The TriLS Approach for Drift-Aware Time-Series Prediction in IIoT Environment
IEEE Transactions on Industrial Informatics(2022)
摘要
This article presents a novel drift-aware approach to multivariate time-series modeling in the nonstationary industrial Internet of Things environments. The three-layered three-state (TriLS) system enables cooperation between the gateway and the cloud toward the timely adjustment of a lightweight predictive model. Concept drift is detected by the cloud with the use of the extended adaptive windowing algorithm that operates on statistics of time sequences tracked by the gateway. This system is geared toward providing accurate predictions of nonstationary industrial processes for intelligent factory automation and safety. The proposed TriLS system is evaluated on records of recurring chemical processes collected at two plants and implemented on a Raspberry Pi board. TriLS achieves a lower prediction error than the reference adaptive schemes while reducing the computational effort and memory requirements for adaptation at the gateway by over 66% and 48%, respectively. It also reduces the volume of shared data between the gateway and the cloud by 40% –72% that is a significant cut on communications overhead.
更多查看译文
关键词
Adaptive windowing (ADWIN),concept drift,fast orthogonal search (FOS),industrial Internet of Things (IIoT),machine learning (ML),time series
AI 理解论文
溯源树
样例
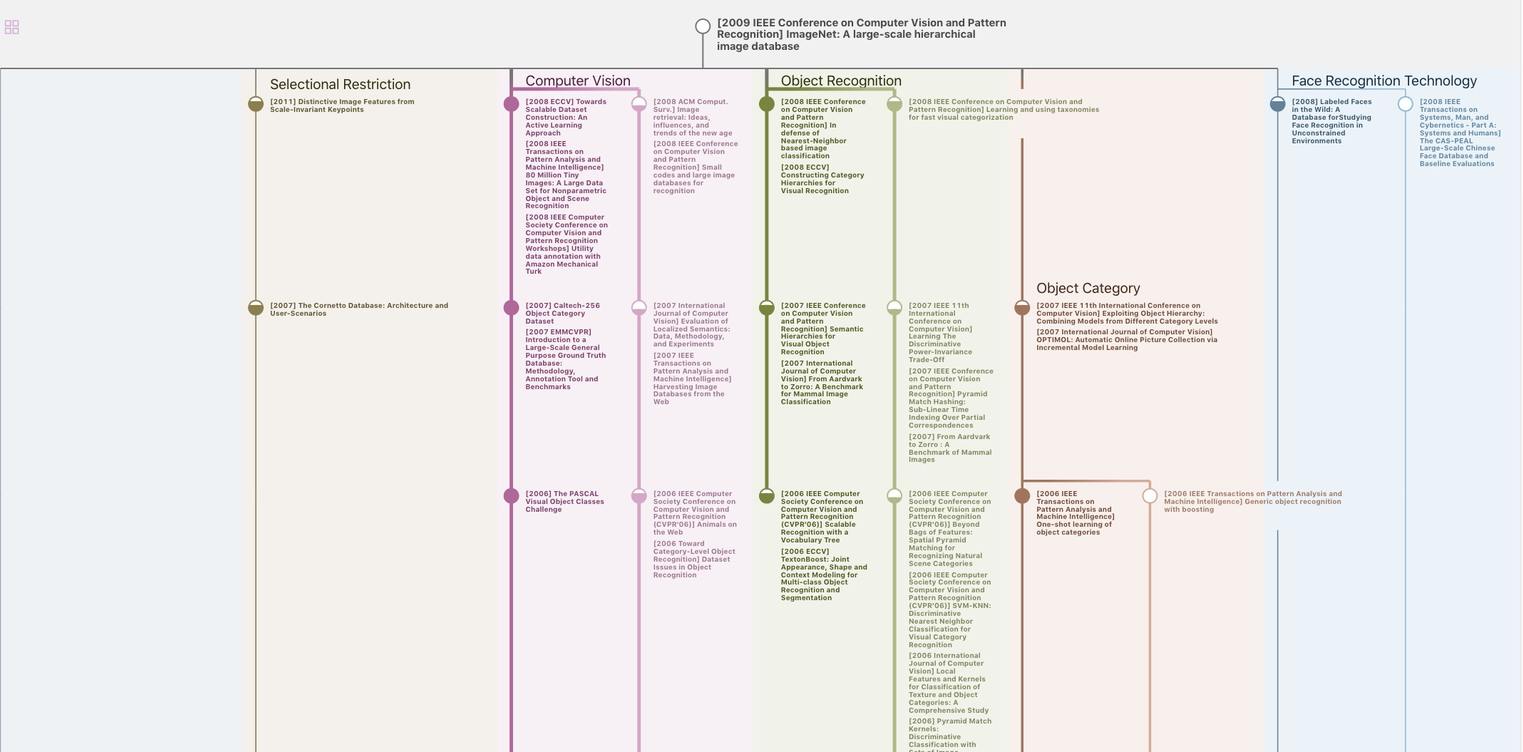
生成溯源树,研究论文发展脉络
Chat Paper
正在生成论文摘要