OnSum: Extractive Single Document Summarization Using Ordered Neuron LSTM
ICIC (2)(2021)
摘要
A growing trend of extractive summarization research is to take the document structure into account which has been shown to correlate with the important contents in a text. However, building complex document structures such as Rhetorical Structure Theory (RST) is time-consuming and requires a large effort to prepare labeled training data. Therefore, how to effectively learn a document structure for summarization remains an open question. Recent findings in the language model area show that the syntactic distance of the basic semantic unit could be used to induce the syntactic structure without any extra labeled data. Inspired by these findings, we propose to extend the basic semantic units from words to sentences and extract the syntactic distance of each sentence in the document by building an ON-LSTM (Ordered Neuron LSTM) based model with the document-level language model objective. We then leverage these syntactic distances to evaluate whether a sentence could be extracted to the summary. Our model achieves state-of-the-art performance in terms of Rouge-1 (48.67) and Rouge-2 (26.32) on the CNN/Daily Mail data set.
更多查看译文
关键词
Extractive summarization,Ordered neuron LSTM,Document structure
AI 理解论文
溯源树
样例
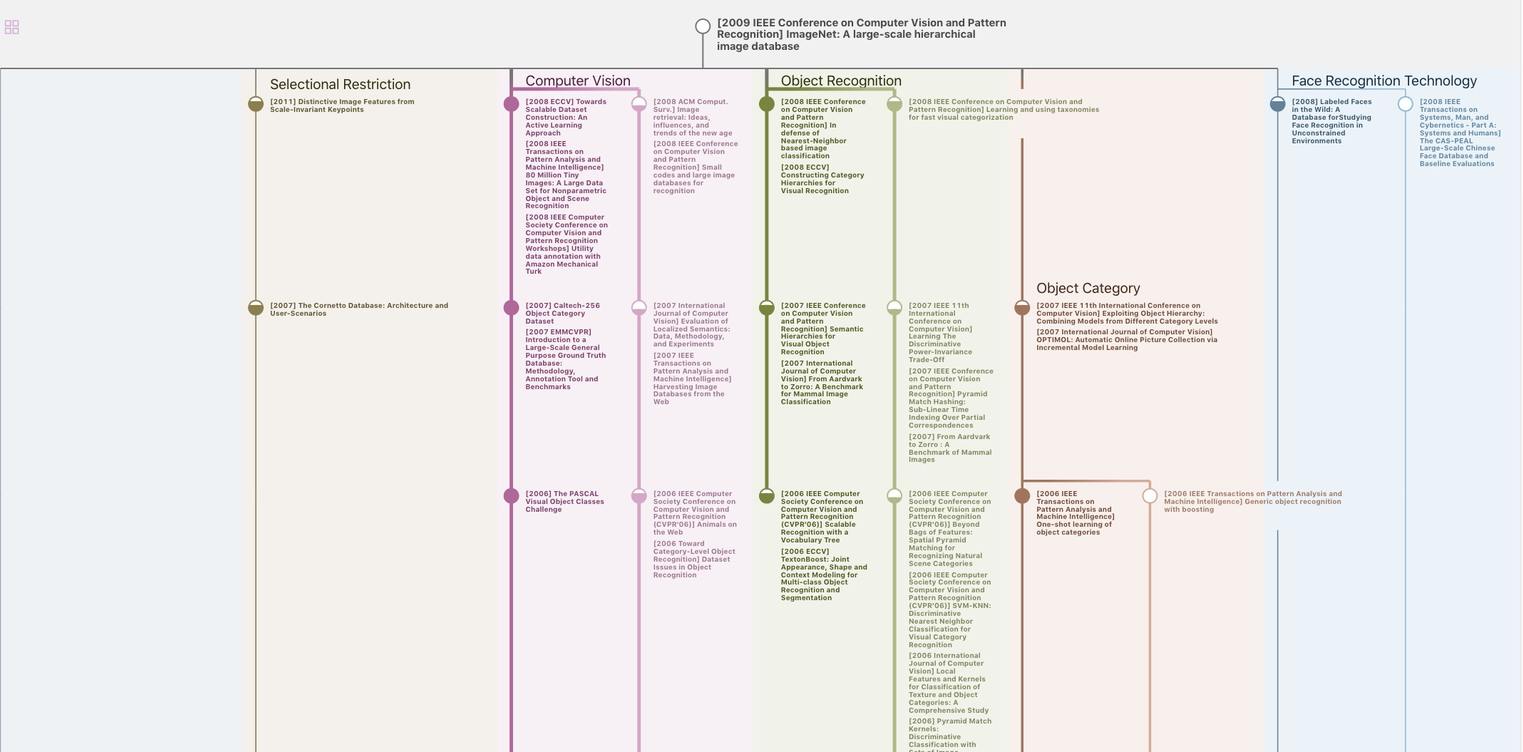
生成溯源树,研究论文发展脉络
Chat Paper
正在生成论文摘要