Deep Performance Factors Analysis for Knowledge Tracing.
AIED (1)(2021)
摘要
Knowledge tracing is the task of dynamically tracking a student's mastery of skills based on their assessment performances and learning-related information (e.g., time spent answering a particular question). Traditional approaches (e.g., Bayesian knowledge tracing [BKT] and performance factors analysis [PFA]), are easy to interpret. Modern approaches (e.g., deep knowledge tracing [DKT] and dynamic key-value memory networks [DKVMN]) usually produce superior performance on certain datasets, but their model complexity causes difficulty in scaling and linking them to existing educational measurement studies. In this paper, we present a simple but effective model, deep performance factors analysis (DPFA) (Source code is available at https://github. com/scott-pu- pennstate/dpfa), to resolve this problem. DPFA consistently outperforms PFA and DKT and has results comparable to those of DKVMN when tested on widely used public datasets. In addition, DPFA's light weight in parameters makes it easy to scale. Finally, we demonstrate a straightforward approach to enhance the base DPFA by incorporating features from the educational measurement literature. The enhanced DPFA showed superior performance than DKVMN.
更多查看译文
关键词
Deep knowledge tracing,Knowledge tracing,Performance factors analysis
AI 理解论文
溯源树
样例
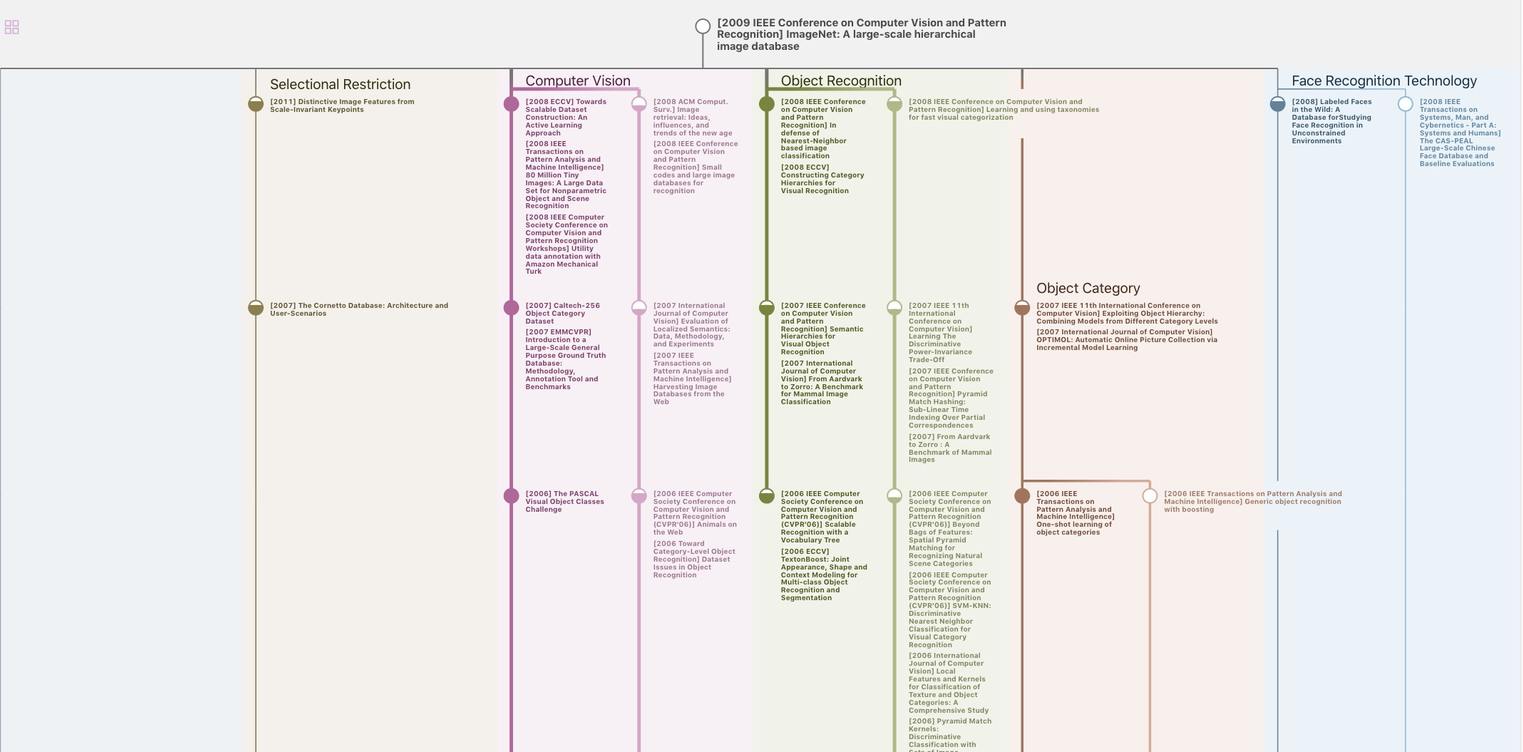
生成溯源树,研究论文发展脉络
Chat Paper
正在生成论文摘要