A Robust Approach for Continuous Interactive Actor-Critic Algorithms.
IEEE Access(2021)
Key words
Continuous interactive reinforcement learning,interactive robust reinforcement learning,reinforcement learning,robust reinforcement learning
AI Read Science
Must-Reading Tree
Example
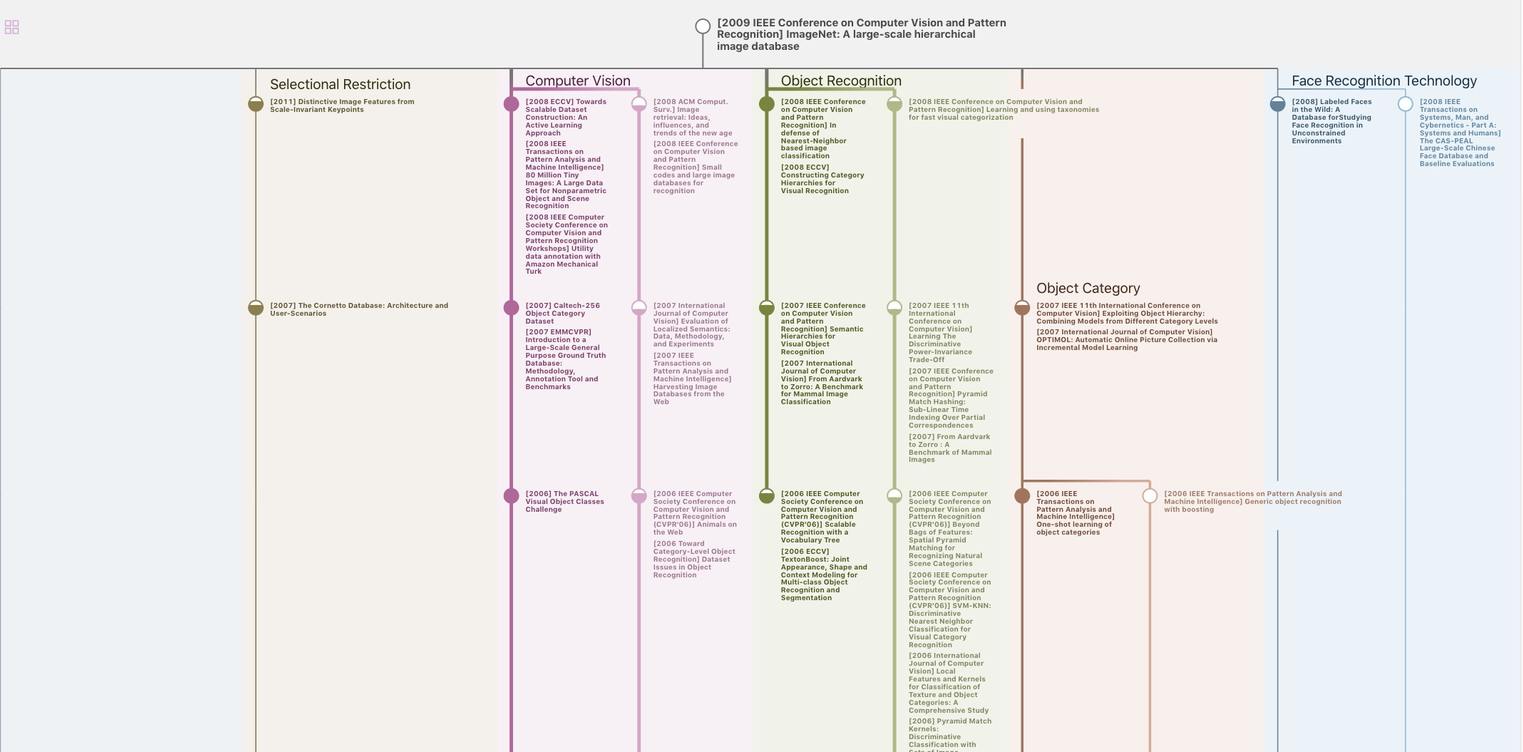
Generate MRT to find the research sequence of this paper
Chat Paper
Summary is being generated by the instructions you defined