Leveraging Redundancy in Attention with Reuse Transformers
ICLR 2022(2022)
Key words
Transformers,attention,redundancy,reuse,efficient
AI Read Science
Must-Reading Tree
Example
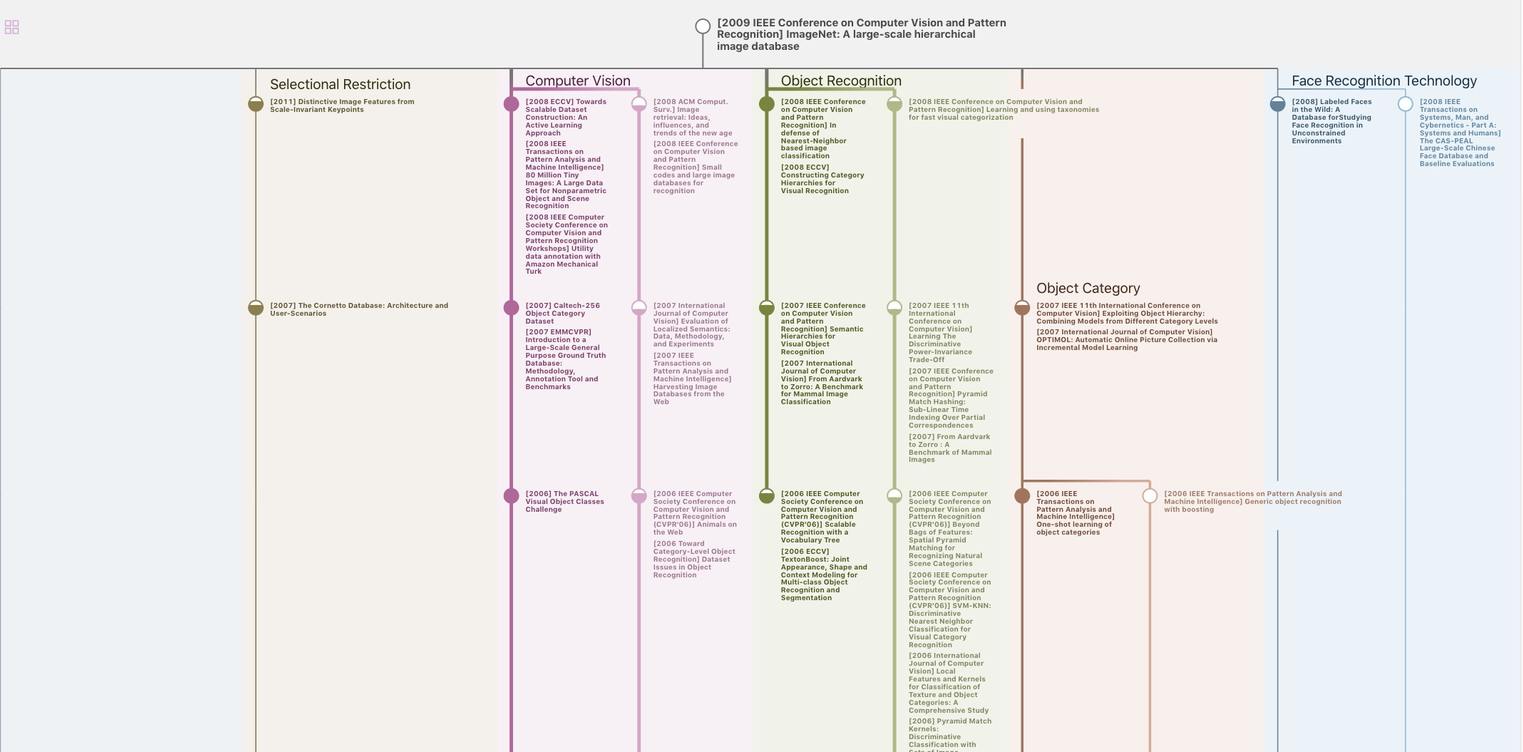
Generate MRT to find the research sequence of this paper
Chat Paper
Summary is being generated by the instructions you defined