Dyn-Backdoor: Backdoor Attack on Dynamic Link Prediction
IEEE Transactions on Network Science and Engineering(2023)
Key words
Dynamic link prediction,backdoor attack,generative adversarial network,gradient exploration
AI Read Science
Must-Reading Tree
Example
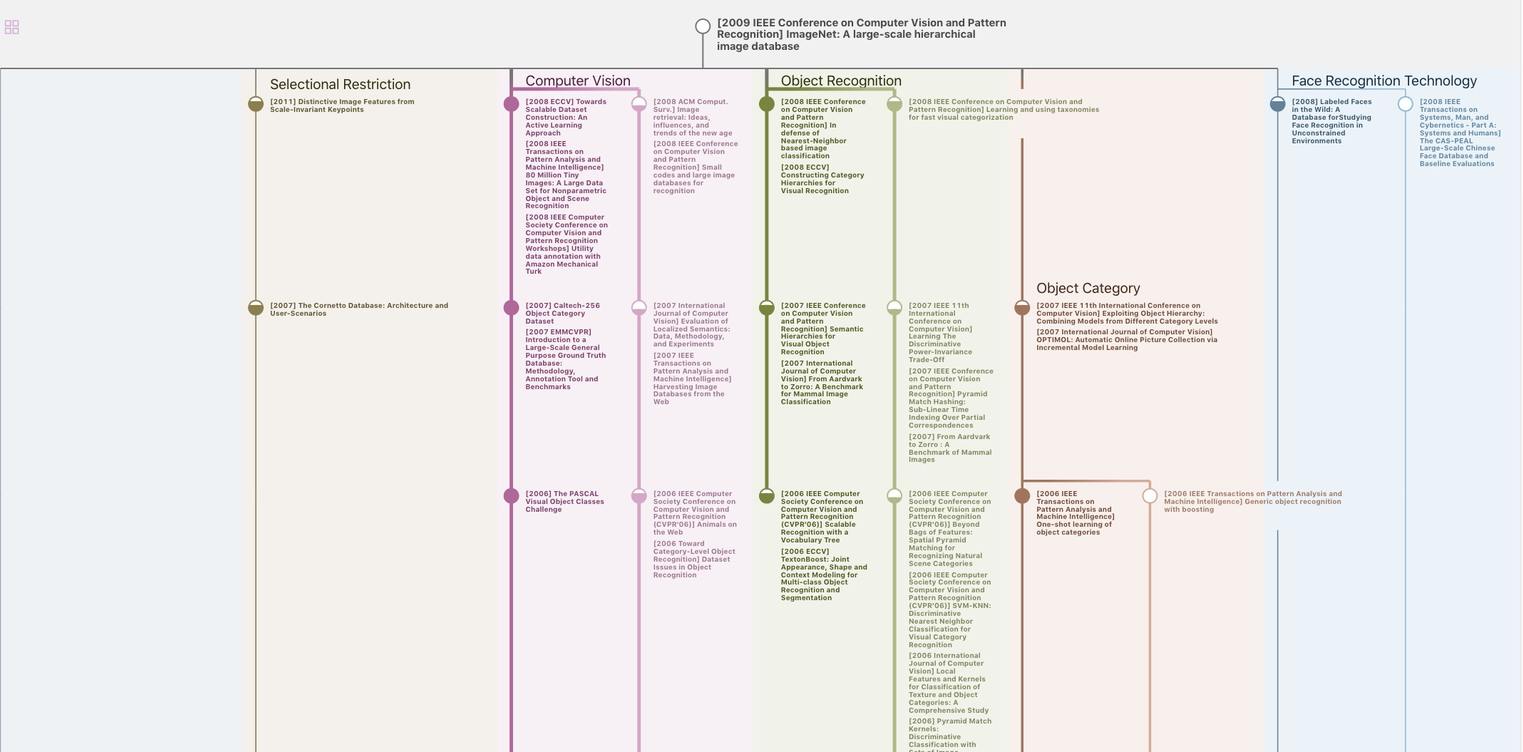
Generate MRT to find the research sequence of this paper
Chat Paper
Summary is being generated by the instructions you defined