Improving Speaker Identification for Shared Devices by Adapting Embeddings to Speaker Subsets
2021 IEEE Automatic Speech Recognition and Understanding Workshop (ASRU)(2021)
关键词
speaker identification,adaptation network,household scoring model,personalization
AI 理解论文
溯源树
样例
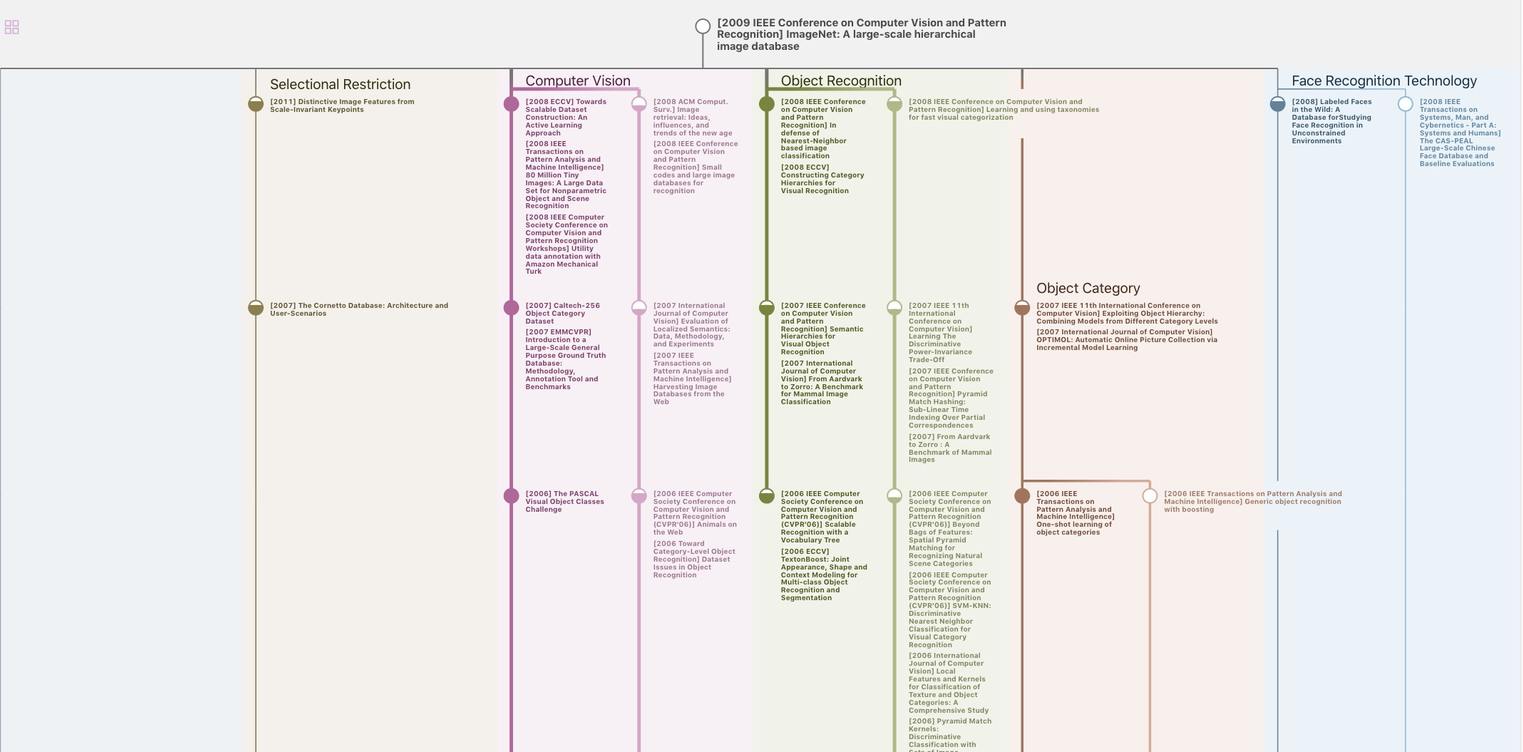
生成溯源树,研究论文发展脉络
Chat Paper
正在生成论文摘要