Quantitative Parametric Mapping of Tissues Properties from Standard Magnetic Resonance Imaging Enabled by Deep Learning
arxiv(2021)
摘要
Magnetic resonance imaging (MRI) offers superior soft tissue contrast and is widely used in biomedicine. However, conventional MRI is not quantitative, which presents a bottleneck in image analysis and digital healthcare. Typically, additional scans are required to disentangle the effect of multiple parameters of MR and extract quantitative tissue properties. Here we investigate a data-driven strategy Q^2 MRI (Qualitative and Quantitative MRI) to derive quantitative parametric maps from standard MR images without additional data acquisition. By taking advantage of the interdependency between various MRI parametric maps buried in training data, the proposed deep learning strategy enables accurate prediction of tissue relaxation properties as well as other biophysical and biochemical characteristics from a single or a few images with conventional T_1/T_2 weighting. Superior performance has been achieved in quantitative MR imaging of the knee and liver. Q^2 MRI promises to provide a powerful tool for a variety of biomedical applications and facilitate the next generation of digital medicine.
更多查看译文
关键词
magnetic resonance imaging,tissues properties,deep learning,quantitative parametric mapping
AI 理解论文
溯源树
样例
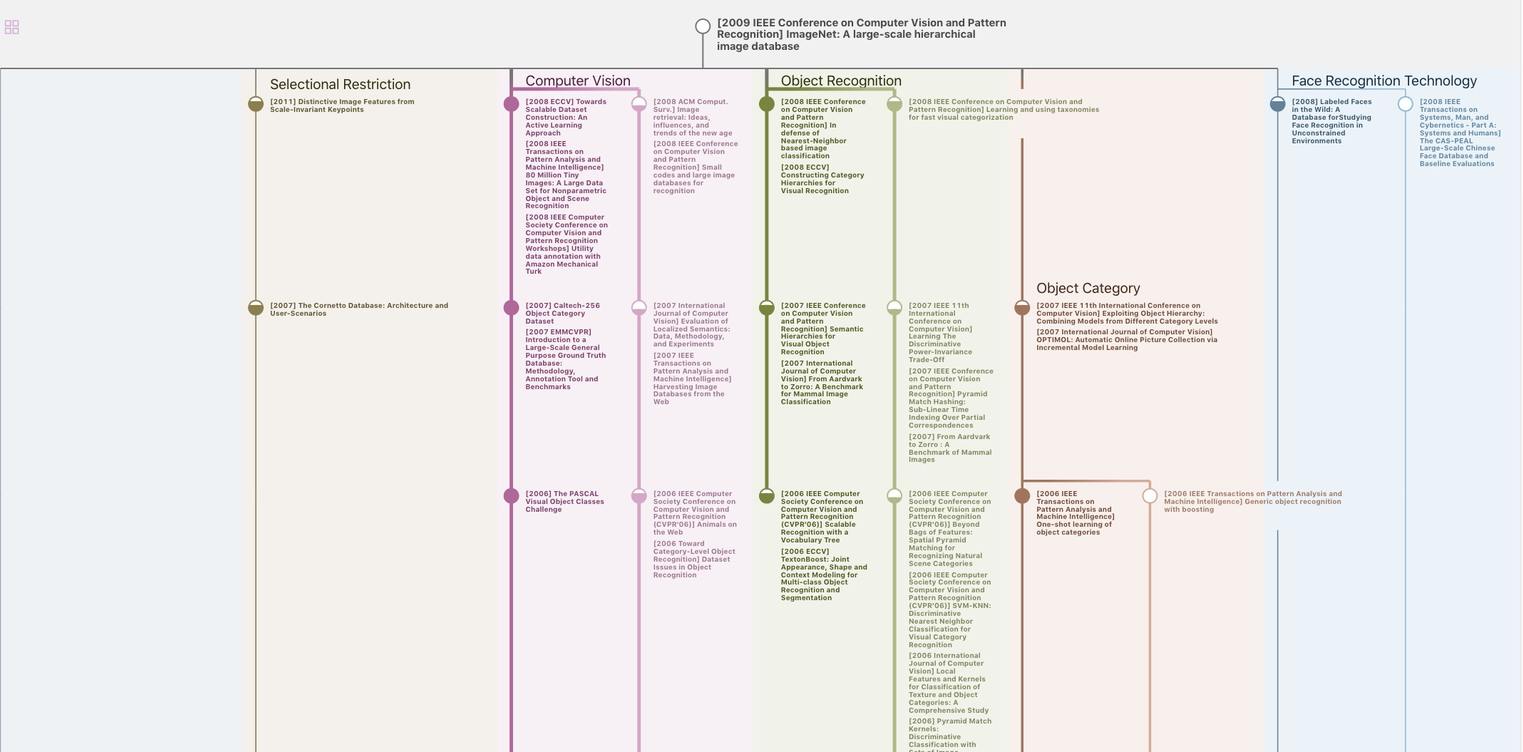
生成溯源树,研究论文发展脉络
Chat Paper
正在生成论文摘要