Unsupervised IoT Fingerprinting Method via Variational Auto-encoder and K-means
IEEE INTERNATIONAL CONFERENCE ON COMMUNICATIONS (ICC 2021)(2021)
摘要
With the rapid growth of the number of IoT devices on the Internet, security problems of IoT devices are becoming more and more serious, which bring more challenges to network administrators. The first task to solve these problems for network administrators is being aware of IoT devices in the network. Previous IoT device identification methods typically use supervised machine learning methods, which require a large amount of labeled sample data. However, it is difficult to obtain a large number of labeled samples effectively. In order to address this problem, we propose an unsupervised IoT device fingerprinting method at the network level, which can effectively cluster IoT devices without labeled samples. We deeply analyze the temporal and spatial dimension characteristics of network traffic, which can adequately reflect the differences between different IoT devices. By using these features, we develop a clustering framework based on variational autoencoder and K-means algorithms. We conduct evaluation experiments on a public dataset including 24 different IoT devices. The experimental results show that our clustering algorithm can achieve accuracy of 86.7% outperforming a k-NN based state-of-art supervised approach.
更多查看译文
关键词
IoT, fingerprinting method, unsupervised
AI 理解论文
溯源树
样例
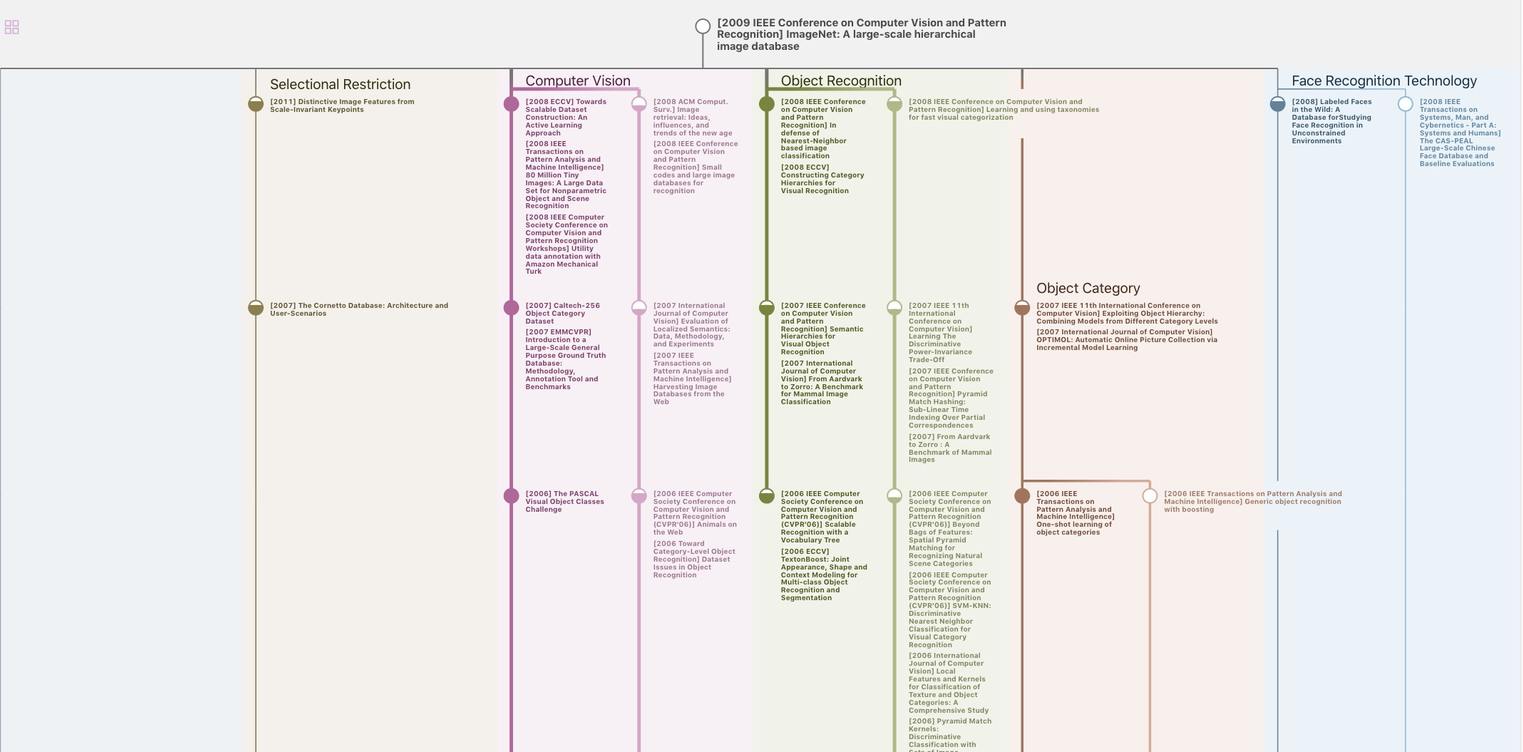
生成溯源树,研究论文发展脉络
Chat Paper
正在生成论文摘要