Machine Learning-Based Long-Term Outcome Prediction In Patients Undergoing Percutaneous Coronary Intervention
CARDIOVASCULAR DIAGNOSIS AND THERAPY(2021)
摘要
Background: Traditional prognostic risk assessment in patients with coronary artery disease undergoing percutaneous coronary intervention (PCI) is based on a limited selection of clinical and imaging findings. Machine learning (ML) can consider a higher number and complexity of variables and may be useful for characterising cardiovascular risk, predicting outcomes, and identifying biomarkers in large population studies. Methods: We prospectively enrolled 9,680 consecutive patients with coronary artery disease who underwent PCI at our institution between January 2013 and December 2013. Clinical features were selected and used to train 6 different ML models (support vector machine, decision tree, random forest, gradient boosting decision tree, neural network, and logistic regression) to predict cardiovascular outcomes, 10-fold cross-validation to evaluate the performance of models. Results: During the 5-year follow-up, 467 (4.82%) patients died. Eighty-seven risk baseline measurements were used to train ML models. Compared with the other models, the random forest model (RF-PCI) exhibited the best performance on predicting all-cause mortality (area under the receiver operating characteristic curve: 0.71 +/- 0.04). Calibration plots demonstrated a slight overprediction for patients using the RF-PCI model (Hosmer-Lemeshow test: P>0.05). The top 15 features related to PCI candidates' long-term prognosis, among which 11 were laboratory measures. Conclusions: ML models improved the prediction of long-term all-cause mortality in patients with coronary artery disease before PCI. The performance of the RF model was better than that of the other models, providing a meaningful stratification.
更多查看译文
关键词
Machine learning (ML), coronary heart disease, percutaneous coronary intervention (PCI), personalised medicine, predictive model
AI 理解论文
溯源树
样例
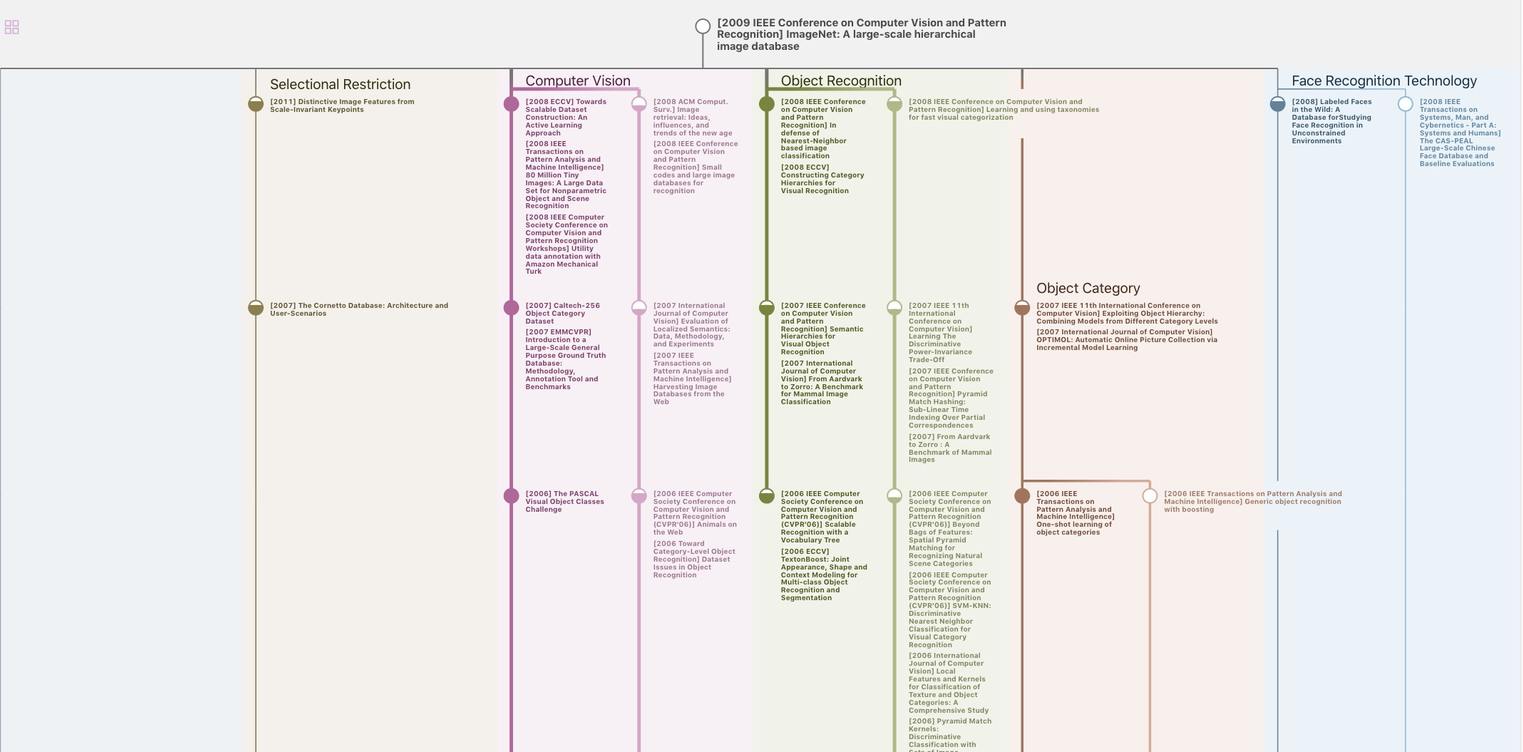
生成溯源树,研究论文发展脉络
Chat Paper
正在生成论文摘要