Huge: An Entropy-Driven Approach To Efficient And Scalable Graph Embeddings
2021 IEEE 37TH INTERNATIONAL CONFERENCE ON DATA ENGINEERING (ICDE 2021)(2021)
摘要
Graph embedding is becoming widely adopted as an efficient way to learn graph representations required to solve graph analytics problems. However, most existing graph embedding methods, owing to computation-efficiency challenges for large-scale graphs, generally employ a one-size-fits-all strategy to extract information, resulting in a large amount of redundant or inaccurate representations. In this work, we propose HuGE, an efficient and scalable graph embedding method enabled by an entropy-driven mechanism. Specifically, HuGE leverages hybrid-property heuristic random walk to capture node features, which considers both node degree and the number of common neighbors in each walking step. More importantly, to guarantee information effectiveness of sampling, HuGE adopts two heuristic methods to decide the random walk length and the number of walks per node, respectively. Extensive experiments on real-world graphs demonstrate that HuGE achieves both efficiency and performance advantages over recent popular graph embedding approaches. For link prediction and multi-label classification, our approach not only offers >10% average gains, but also exhibits 22 x -126 x speedup compared with existing sampling-based methods.
更多查看译文
关键词
Graph embedding, Efficiency, Scalability, Entropy-driven
AI 理解论文
溯源树
样例
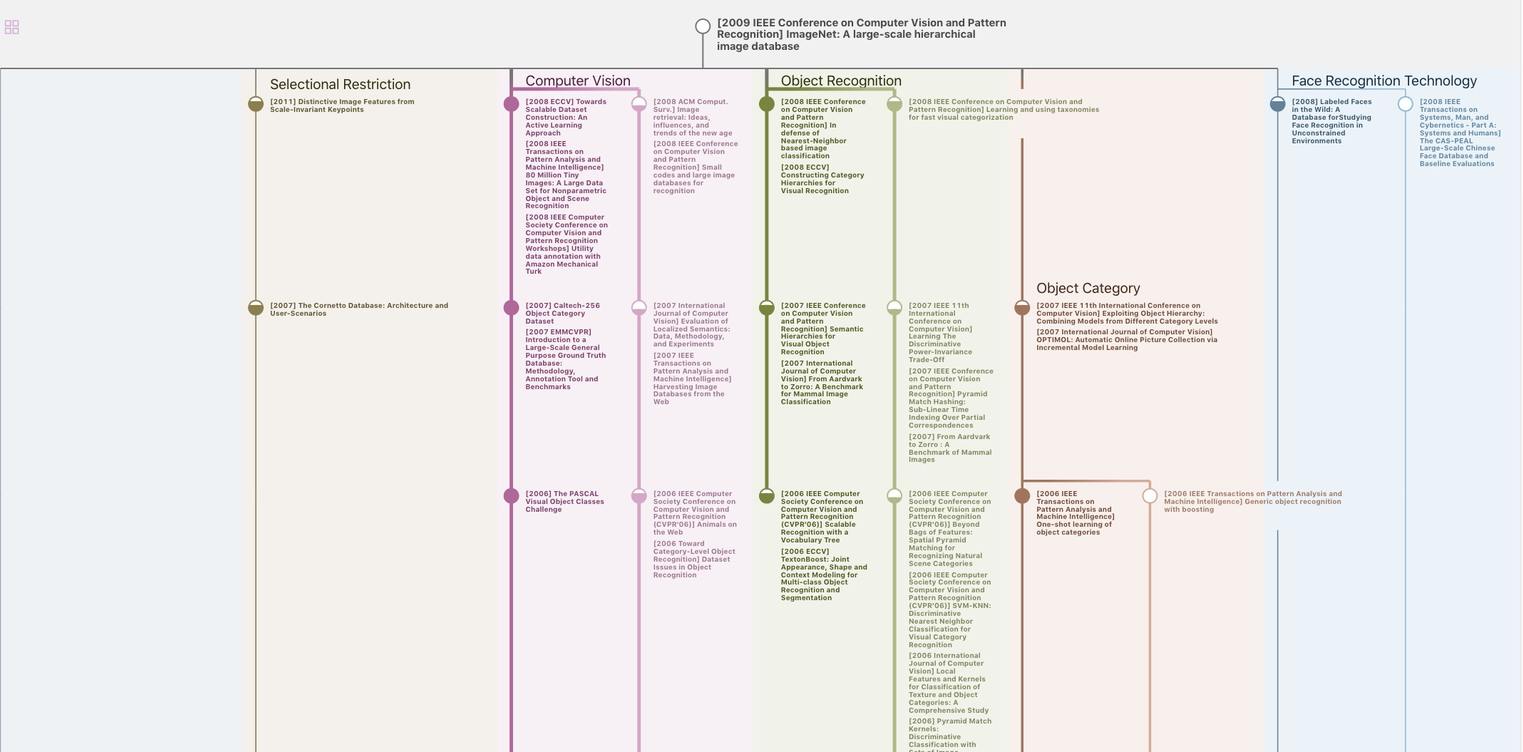
生成溯源树,研究论文发展脉络
Chat Paper
正在生成论文摘要