An Optimal Linear Transformation for Data Assimilation
JOURNAL OF ADVANCES IN MODELING EARTH SYSTEMS(2022)
关键词
data assimilation,kalman filter,covariance localization,linear transformation,canonical correlation analysis
AI 理解论文
溯源树
样例
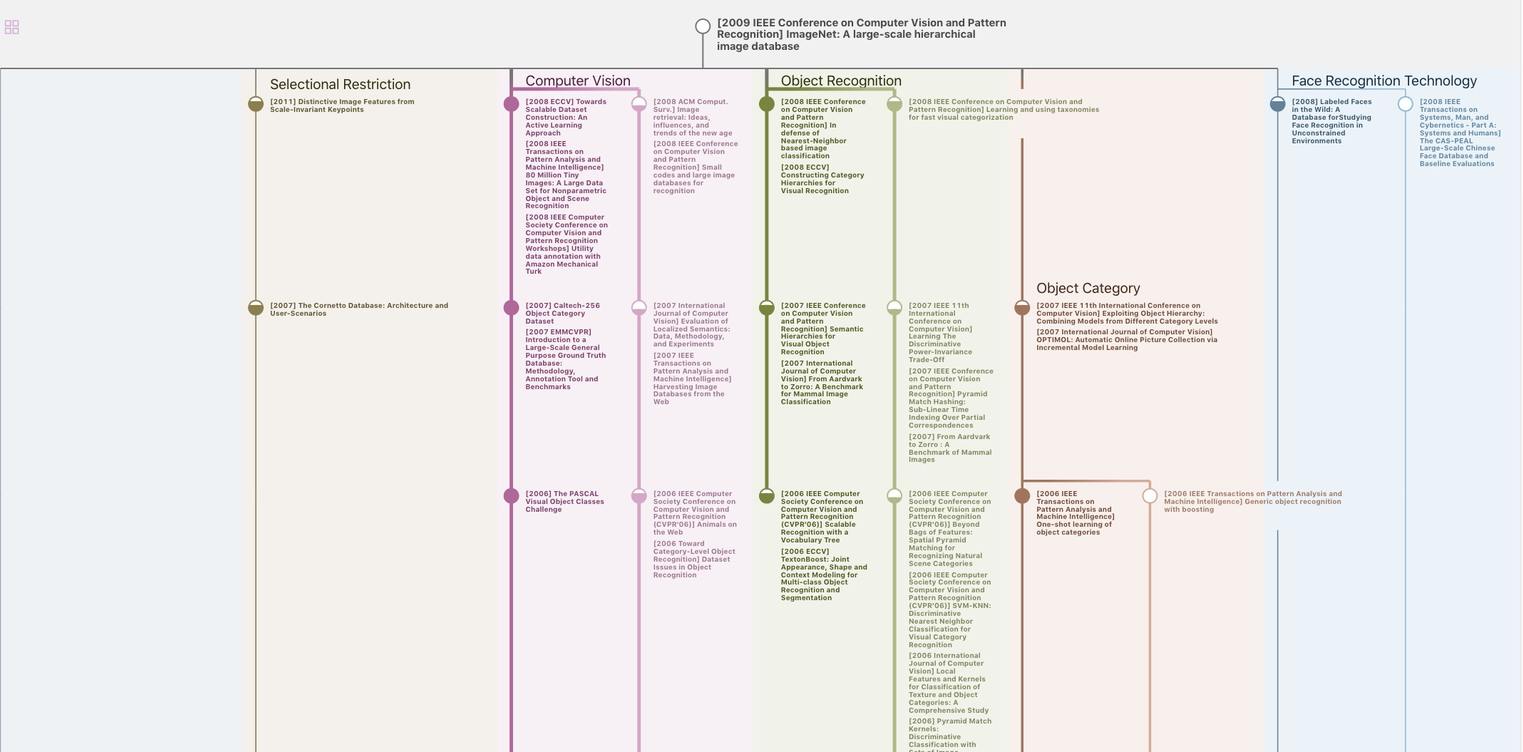
生成溯源树,研究论文发展脉络
Chat Paper
正在生成论文摘要