Screening Patients With Early Stage Parkinson'S Disease Using A Machine Learning Technique: Measuring The Amount Of Iron In The Basal Ganglia
APPLIED SCIENCES-BASEL(2020)
摘要
The purpose of this study was to determine whether a support vector machine (SVM) model based on quantitative susceptibility mapping (QSM) can be used to differentiate iron accumulation in the deep grey matter of early Parkinson's disease (PD) patients from healthy controls (HC) and Non-Motor Symptoms Scale (NMSS) scores in early PD patients. QSM values on magnetic resonance imaging (MRI) were obtained for 24 early PD patients and 27 age-matched HCs. The mean QSM values in deep grey matter areas were used to construct SVM and logistic regression (LR) models to differentiate between early PD patients and HCs. Additional SVM and LR models were constructed to differentiate between low and high NMSS scores groups. A paired t-test was used to assess the classification results. For the differentiation between early PD patients and HCs, SVM had an accuracy of 0.79 +/- 0.07, and LR had an accuracy of 0.73 +/- 0.03 (p = 0.027). SVM for NMSS classification had a fairly high accuracy of 0.79 +/- 0.03, while LR had 0.76 +/- 0.04. An SVM model based on QSM offers competitive accuracy for screening early PD patients and evaluates non-motor symptoms, which may offer clinicians the ability to assess the progression of motor symptoms in the patient population.
更多查看译文
关键词
Parkinson’, s disease, machine learning, support vector machine, quantitative susceptibility mapping, nonmotor symptom
AI 理解论文
溯源树
样例
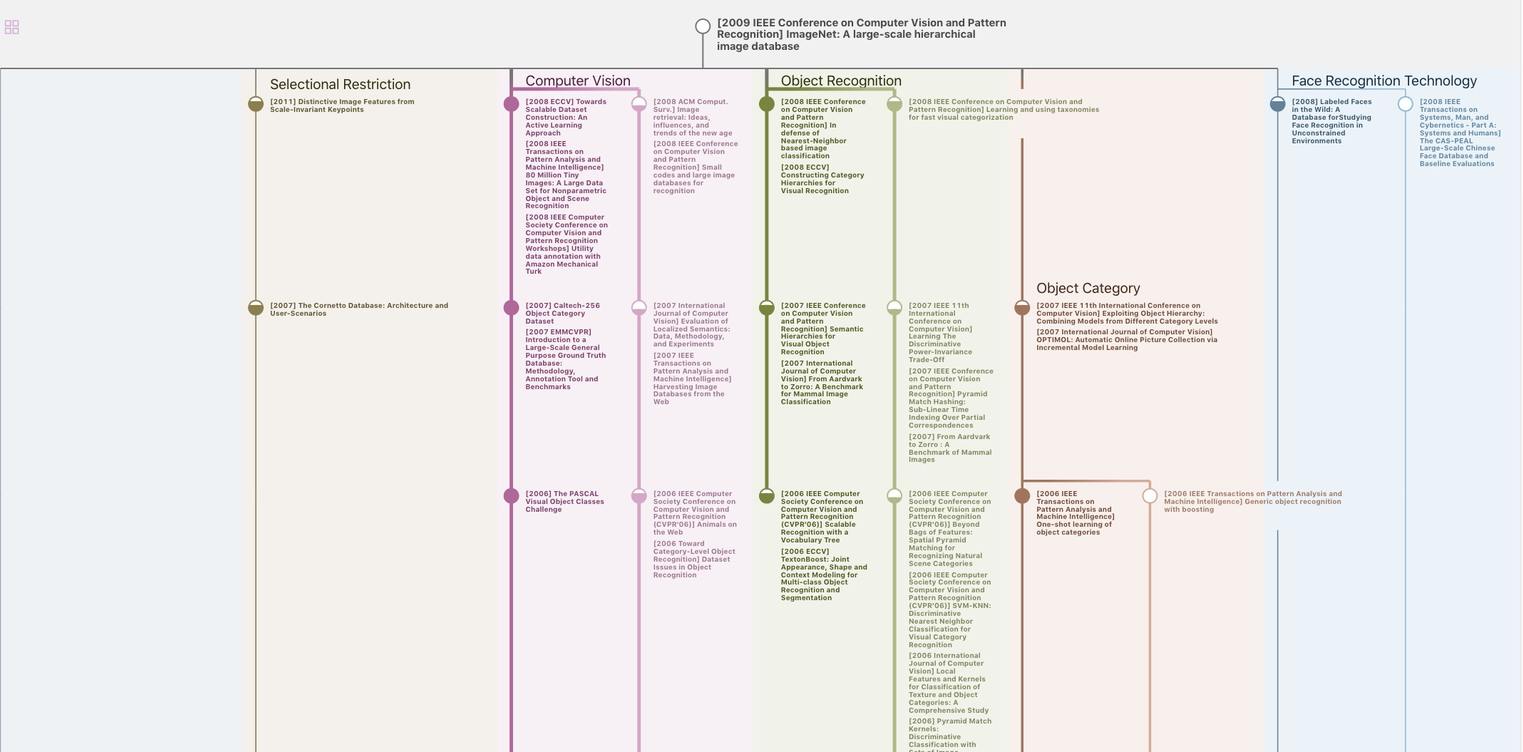
生成溯源树,研究论文发展脉络
Chat Paper
正在生成论文摘要