CaptorX: A Class-Adaptive Convolutional Neural Network Reconfiguration Framework
IEEE Transactions on Computer-Aided Design of Integrated Circuits and Systems(2022)
摘要
Nowadays, the evolution of deep learning and cloud service significantly promotes neural network-based mobile applications. Although intelligent and prolific, those applications still lack certain flexibility: for classification tasks, neural networks are generally trained with vast classification targets to cover various utilization contexts. However, only partial classes are practically inferred due to individual mobile user preference and application specificity, which causes unnecessary computation consumption. Thus, we proposed
CaptorX
—a class-adaptive convolutional neural network (CNN) reconfiguration framework to adaptively prune convolutional filters associated with unneeded classes.
CaptorX
can reconfigure a pretrained full-class CNN model into class-specific lightweight models based on the visualization analysis of convolutional filters’ exclusive functionality for a single class. These lightweight models can be directly deployed to mobile devices without the retraining cost of traditional pruning-based reconfiguration. Furthermore, we can apply the
CaptorX
framework into a distributed collaboration setting. With dedicated local training regulation and collaborative aggregation schemes, the class-adaptive models on individual mobile devices can further contribute back to the central full-class model. Experiments on representative CNNs and image classification datasets show that,
CaptorX
can reduce the CNN computation workload up to 50.22% and save 46.58% energy consumption for varied local devices, meanwhile improving accuracy for their targeted classes with better task focus. With our distributed collaboration paradigm,
CaptorX
also provides further potential to enhance the central model accuracy, while reducing up to 37.58% communication cost compared to traditional distributed learning methods.
更多查看译文
关键词
Convolutional neural network (CNN) reconfiguration,CNN visualization,distributed learning,mobile computing
AI 理解论文
溯源树
样例
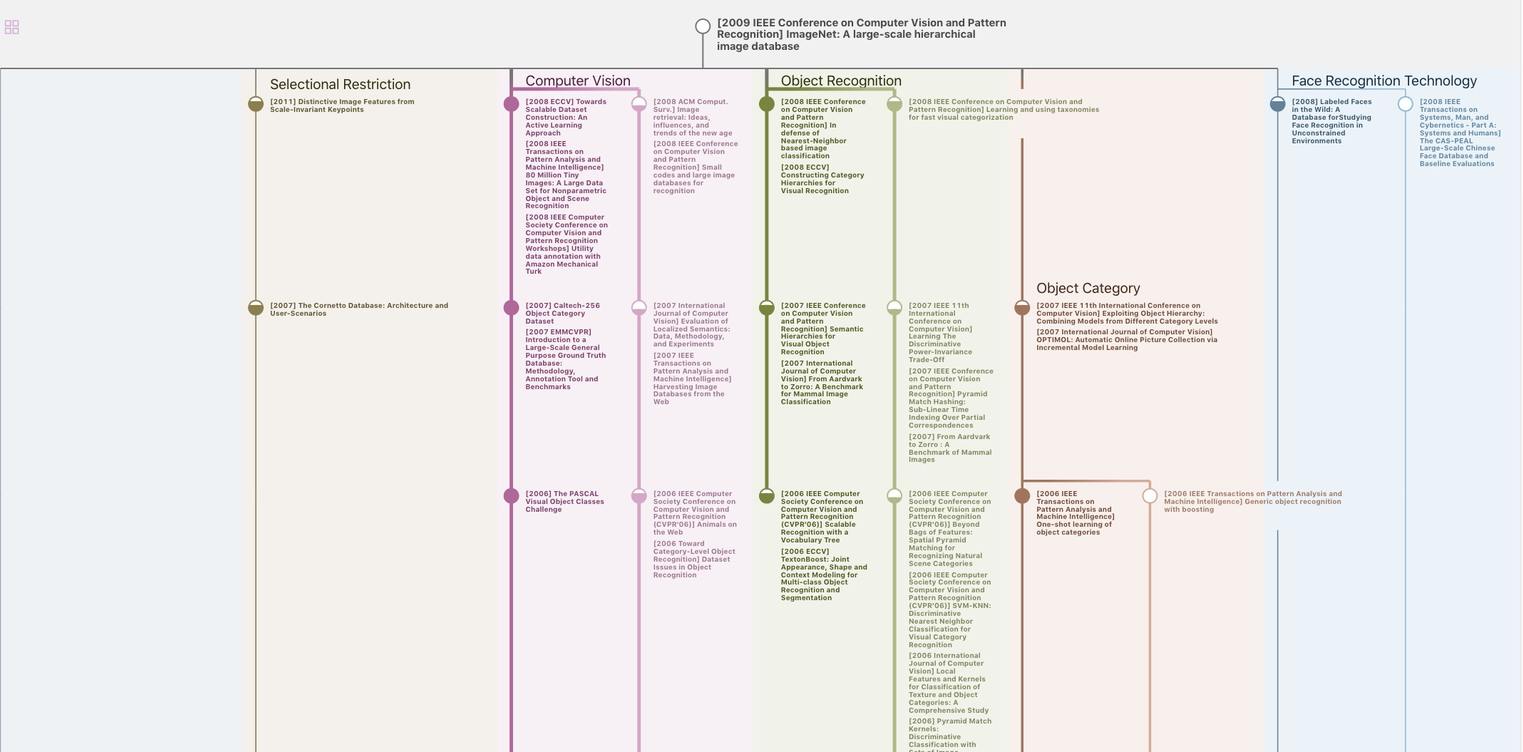
生成溯源树,研究论文发展脉络
Chat Paper
正在生成论文摘要