Softmax with Regularization: Better Value Estimation in Multi-Agent Reinforcement Learning
arXiv (Cornell University)(2021)
AI Read Science
Must-Reading Tree
Example
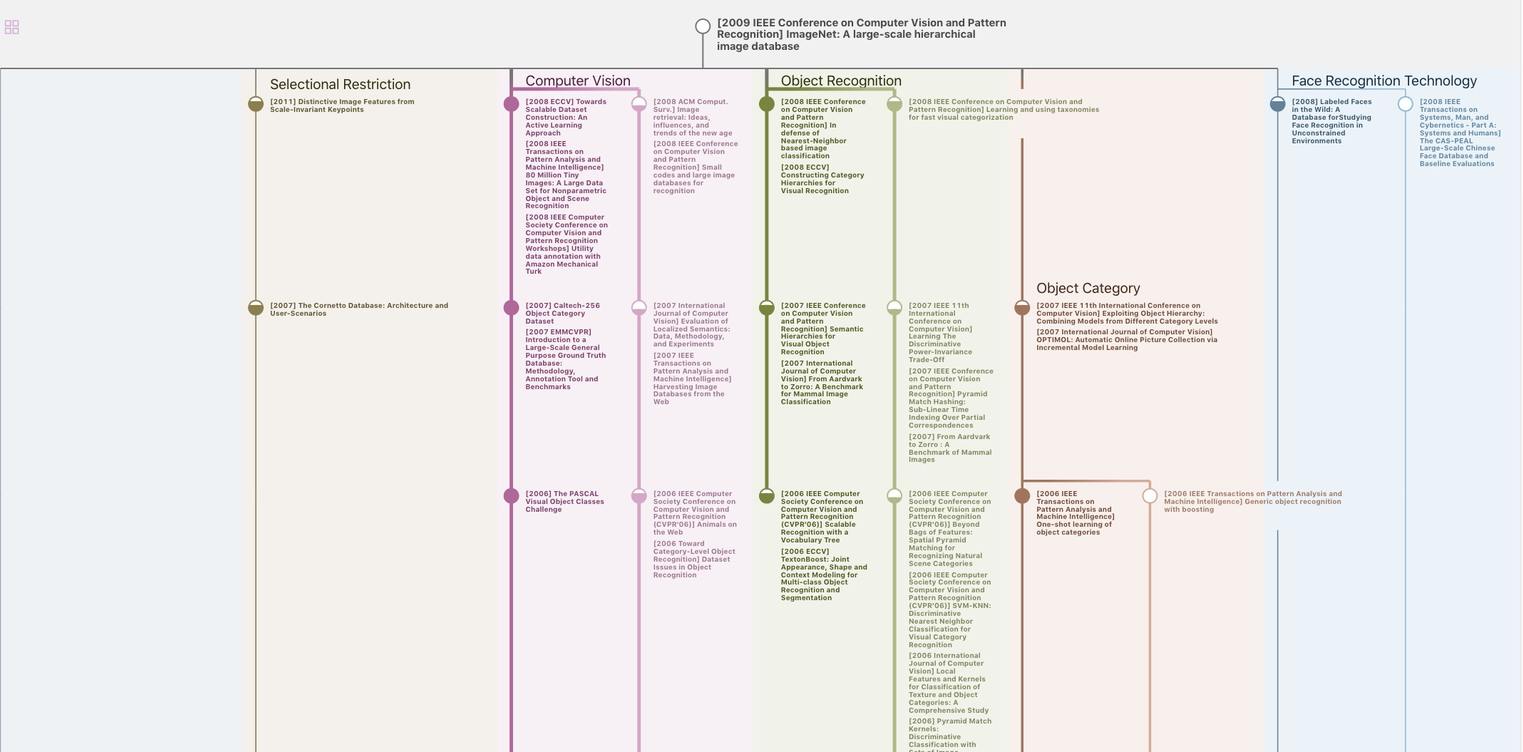
Generate MRT to find the research sequence of this paper
Chat Paper
Summary is being generated by the instructions you defined