An Energy-efficient Multi-core Restricted Boltzmann Machine Processor with On-chip Bio-plausible Learning and Reconfigurable Sparsity.
2020 IEEE Asian Solid-State Circuits Conference (A-SSCC)(2020)
关键词
RBM,multi-core processor,on-chip learning
AI 理解论文
溯源树
样例
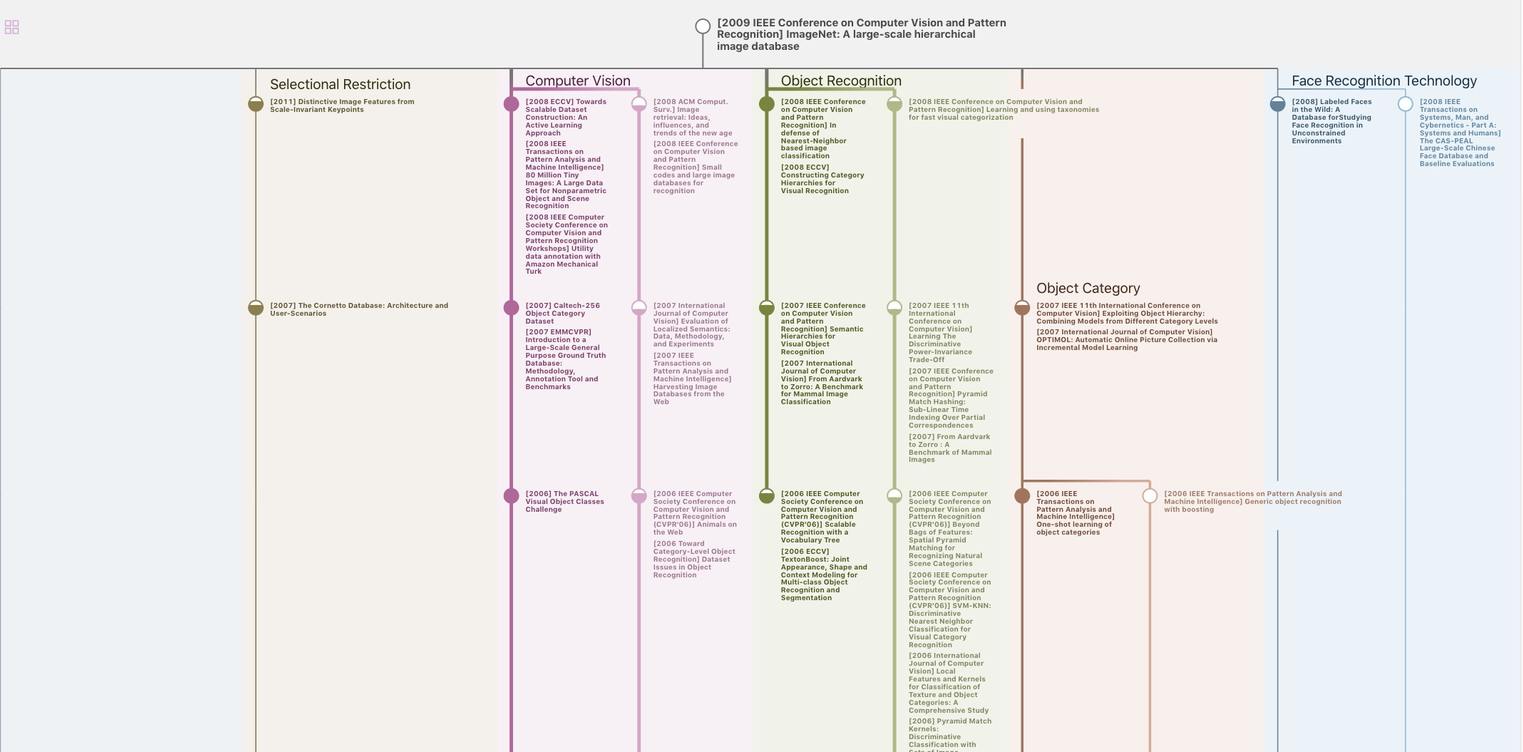
生成溯源树,研究论文发展脉络
Chat Paper
正在生成论文摘要