Cluster Analysis of Coronavirus Sequences using Computational Sequence Descriptors: With Applications to SARS, MERS and SARS-CoV-2 (CoVID-19)
CURRENT COMPUTER-AIDED DRUG DESIGN(2021)
摘要
Introduction: Coronaviruses comprise a group of enveloped, positive-sense single -s-tranded RNA viruses that infect humans as well as a wide range of animals. The study was per -formed on a set of 573 sequences belonging to SARS, MERS and SARS-CoV-2 (CoVID-19) virus -es. The sequences were represented with alignment-free sequence descriptors and analyzed with different chemometric methods: Euclidean/Mahalanobis distances, principal component analysis and self-organizing maps (Kohonen networks). We report the cluster structures of the data. The se-quences are well-clustered regarding the type of virus; however, some of them show the tendency to belong to more than one virus type. Background: This is a study of 573 genome sequences belonging to SARS, MERS and SARS--CoV-2 (CoVID-19) coronaviruses. Objectives: The aim was to compare the virus sequences, which originate from different places around the world. Methods: The study used alignment free sequence descriptors for the representation of sequences and chemometric methods for analyzing clusters. Results: Majority of genome sequences are clustered with respect to the virus type, but some of them are outliers. Conclusion: We indicate 71 sequences, which tend to belong to more than one cluster.
更多查看译文
关键词
SARS-CoV-2 (CoVID-19), SARS, MERS, mathematical representation of sequences, clustering, Euclidean distance, Mahalanobis distance, principal component analysis, alignment-free sequenc descriptors
AI 理解论文
溯源树
样例
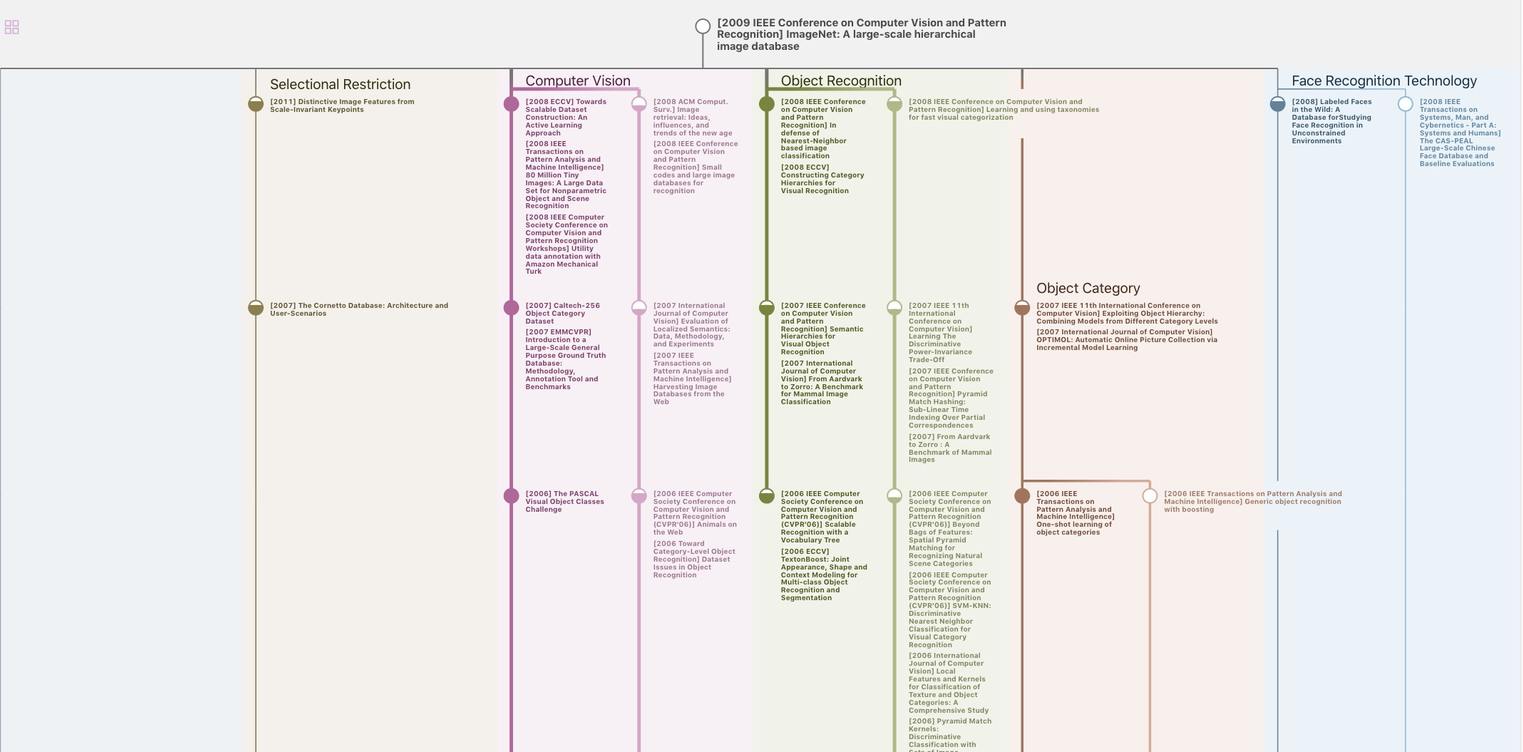
生成溯源树,研究论文发展脉络
Chat Paper
正在生成论文摘要