Constrained Probabilistic Movement Primitives for Robot Trajectory Adaptation
IEEE Transactions on Robotics(2022)
摘要
Placing robotsoutside controlled conditions requires versatile movement representations that allow robots to learn new tasks and adapt them to environmental changes. The introduction of obstacles or the placement of additional robots in the workspace and the modification of the joint range due to faults or range-of-motion constraints are typical cases, where the adaptation capabilities play a key role for safely performing the robot’s task. Probabilistic movement primitives (ProMPs) have been proposed for representing adaptable movement skills, which are modeled as Gaussian distributions over trajectories. These are analytically tractable and can be learned from a small number of demonstrations. However, both the original ProMP formulation and the subsequent approaches only provide solutions to specific movement adaptation problems, e.g., obstacle avoidance, and a generic, unifying, probabilistic approach to adaptation is missing. In this article, we develop a generic probabilistic framework for adapting ProMPs. We unify previous adaptation techniques, for example, various types of obstacle avoidance, via-points, and mutual avoidance, in one single framework and combine them to solve complex robotic problems. Additionally, we derive novel adaptation techniques such as temporally unbound via-points and mutual avoidance. We formulate adaptation as a constrained optimization problem, where we minimize the Kullback–Leibler divergence between the adapted distribution and the distribution of the original primitive,while we constrain the probability mass associated with undesired trajectories to be low. We demonstrate our approach on several adaptation problems on simulated planar robot arms and seven-degree-of-freedom Franka Emika robots in a dual-robot-arm setting.
更多查看译文
关键词
Constrained optimization,collision avoidance,learning from demonstrations,movement primitives,probabilistic models,robot learning,trajectory optimization
AI 理解论文
溯源树
样例
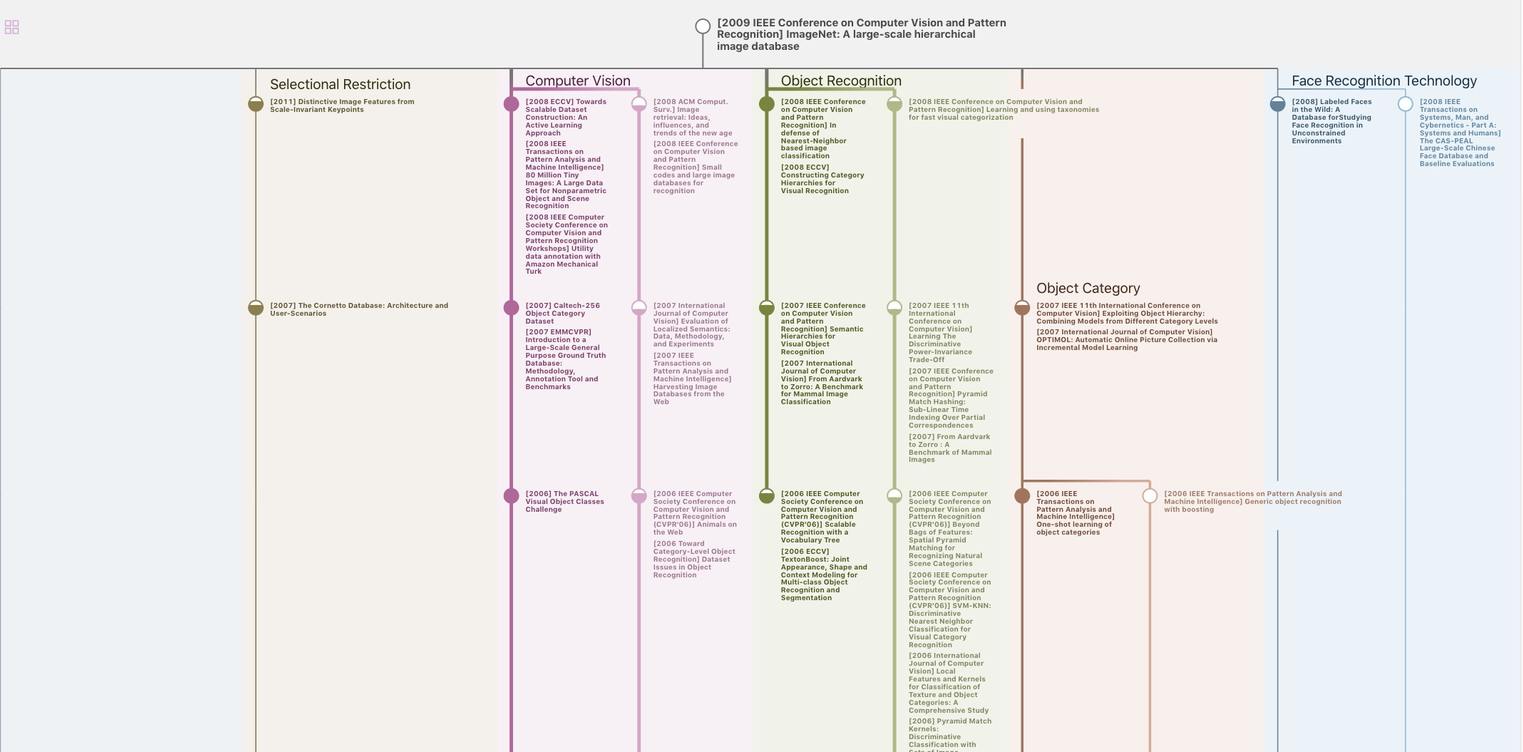
生成溯源树,研究论文发展脉络
Chat Paper
正在生成论文摘要