Predicting Neural Deterioration in Patients with Alzheimer’s Disease Using a Convolutional Neural Network
IEEE International Conference on Bioinformatics and Biomedicine(2020)
关键词
deep learning,Alzheimer’s disease,disease progression model,convolutional neural network,small data
AI 理解论文
溯源树
样例
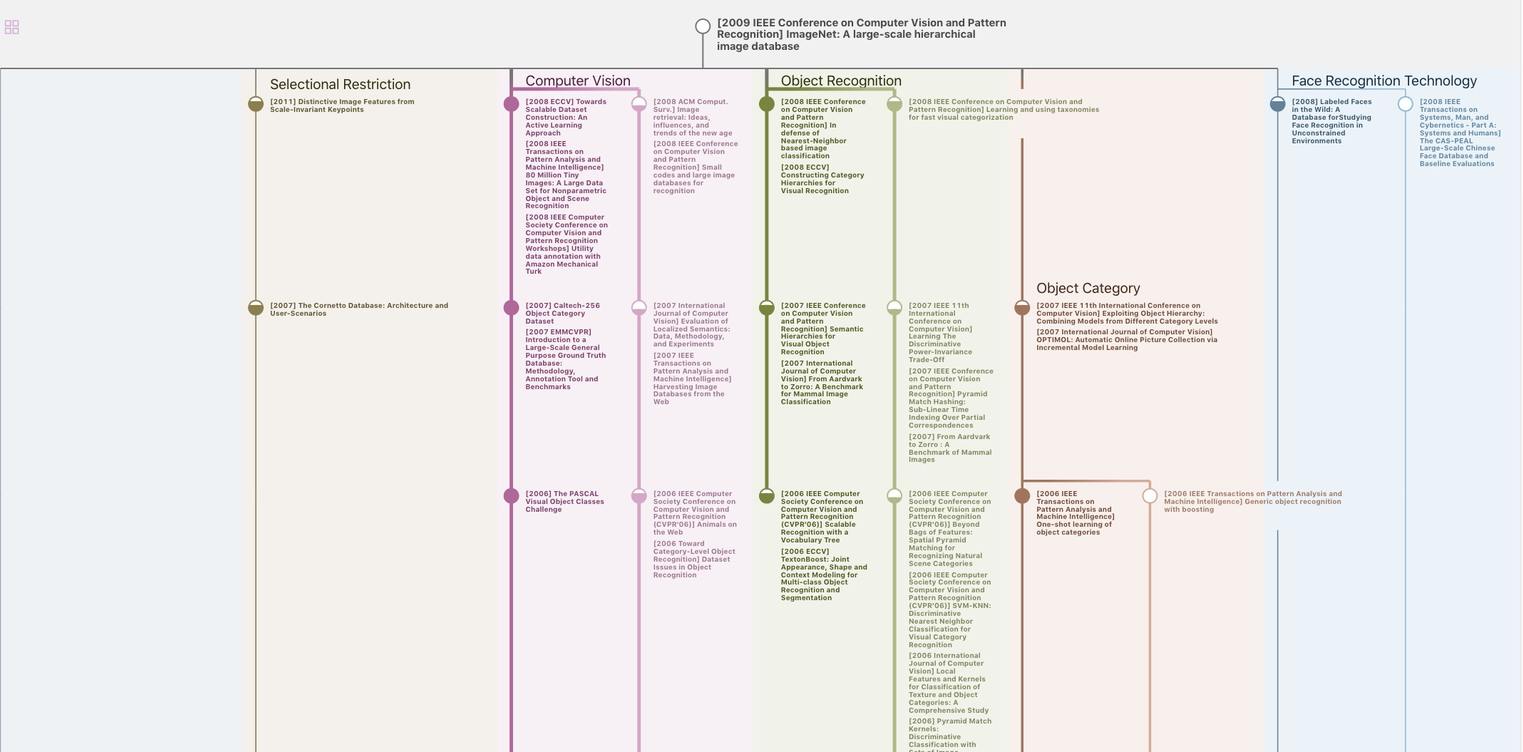
生成溯源树,研究论文发展脉络
Chat Paper
正在生成论文摘要