Gender Stereotypes in Natural Language: Word Embeddings Show Robust Consistency Across Child and Adult Language Corpora of More Than 65 Million Words
PSYCHOLOGICAL SCIENCE(2021)
关键词
collective representations,gender stereotypes,machine learning,natural-language processing,word embeddings,open data,open materials
AI 理解论文
溯源树
样例
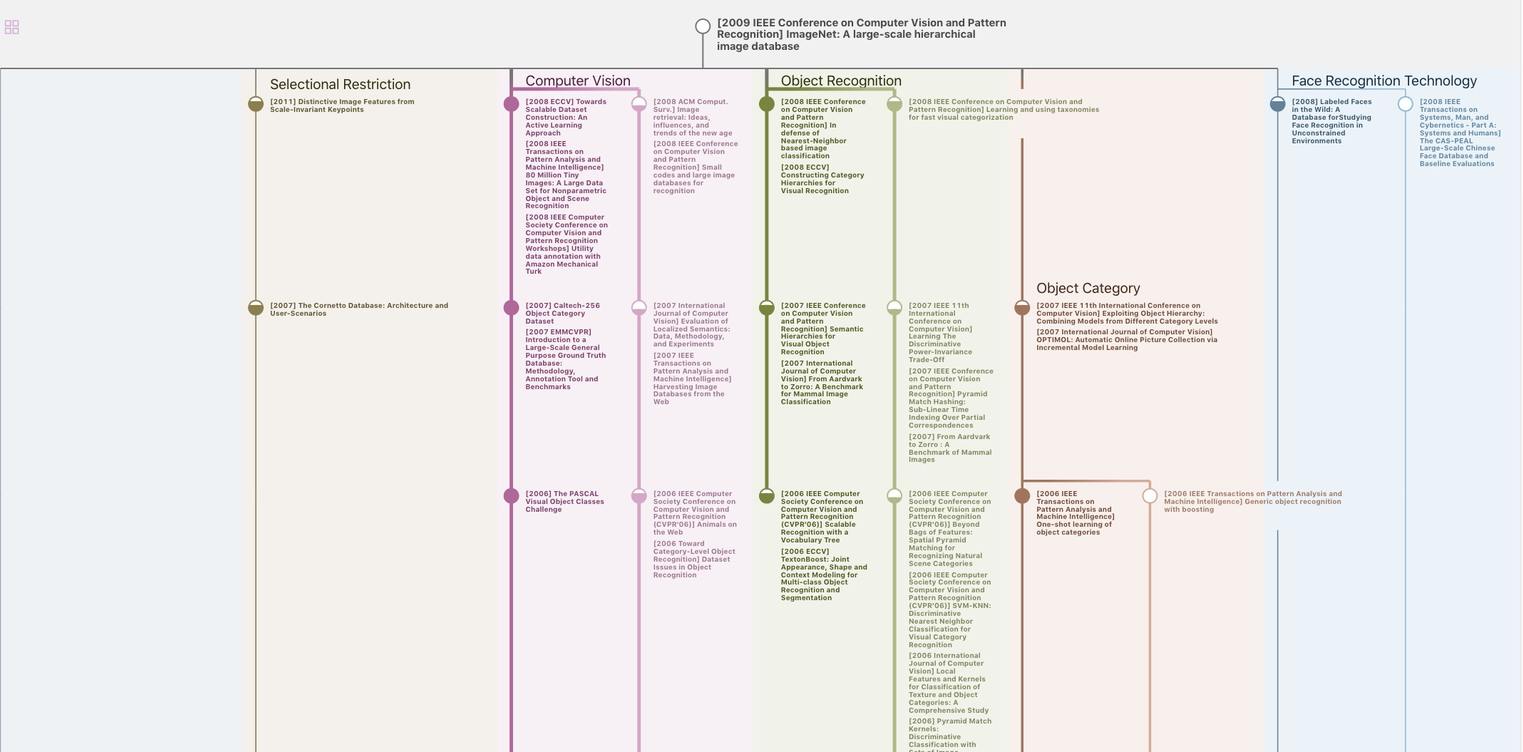
生成溯源树,研究论文发展脉络
Chat Paper
正在生成论文摘要