A relaxed model selection method for Duffing oscillator identification
2020 28th Mediterranean Conference on Control and Automation (MED)(2020)
摘要
This study presents a relaxed model selection procedure based on the sparse regression system identification method for Duffing oscillator identification. A two-stage relaxation procedure is presented. In a first stage an elastic net optimizer underpins the sparse regression that enables to explore the possible models. The subsequent stage employs the Akaike information criteria that employs the one standard error rule (1-SE) to select the appropriate model. We study the effect of relaxation in both stages, i.e. providing more exploration capabilities in the regression and the selection, by applying the two-stage methodology on experimental data collected on a mechanical Duffing oscillator setup. Our analysis shows that relaxation is advantageous when dealing with noisy experimental data and allows to find model structures and associated parameter values in the mechatronic Duffing oscillator. Results show that relaxation is pivotal when dealing with noisy experimental data and that the methodology possesses the capability to find model structures and associated parameter values of a Duffing oscillator. The presented results can be of benefit when identifying the system behavior of other mechatronic systems that exhibit complex, chaotic behavior that is difficult to model starting from first principles.
更多查看译文
关键词
Duffing oscillator,system identification,model selection,Sparse regression,information criteria
AI 理解论文
溯源树
样例
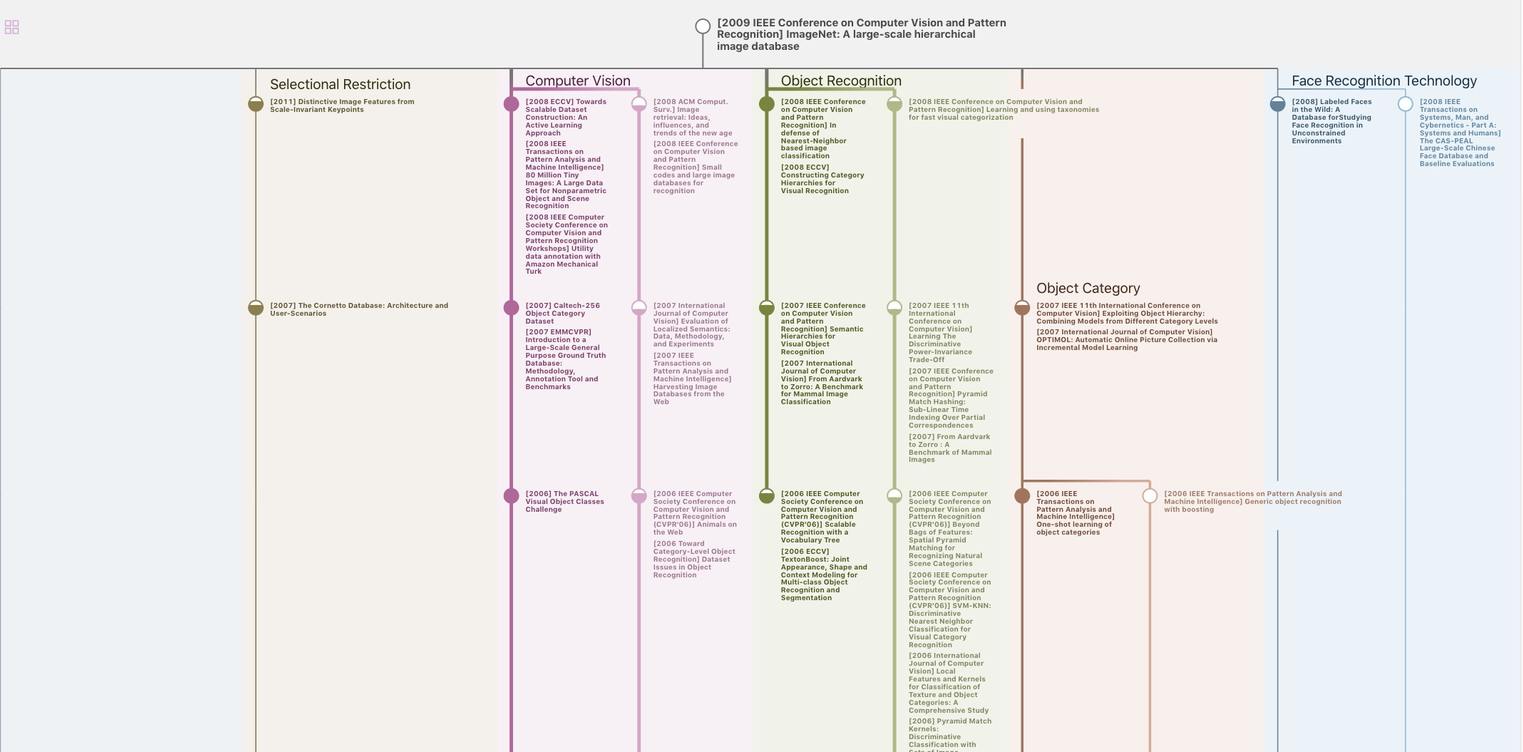
生成溯源树,研究论文发展脉络
Chat Paper
正在生成论文摘要