Deep Semi-Supervised Embedded Clustering (DSEC) for Stratification of Heart Failure Patients
CoRR(2020)
AI Read Science
Must-Reading Tree
Example
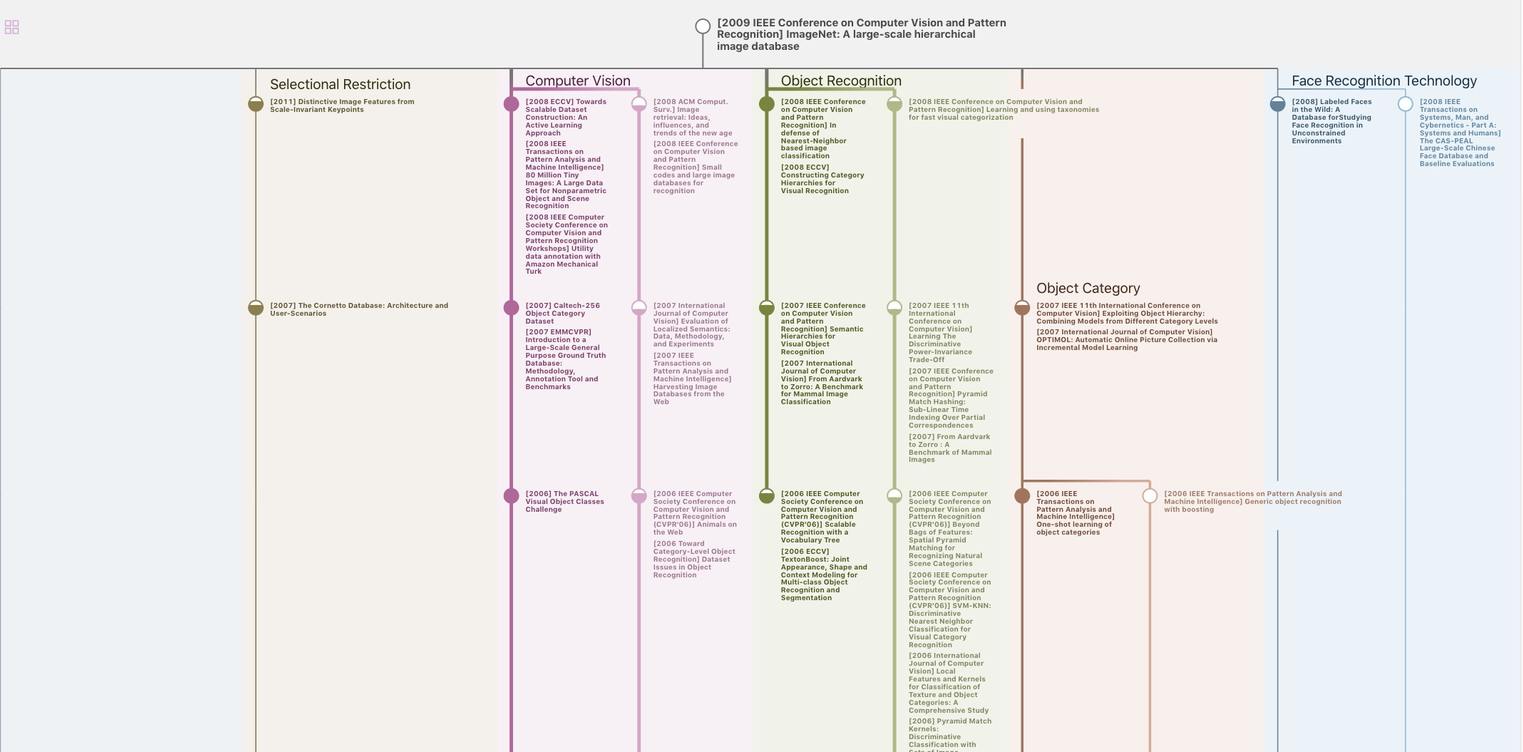
Generate MRT to find the research sequence of this paper
Chat Paper
Summary is being generated by the instructions you defined