A New Data Augmentation Method Based on Local Image Warping for Medical Image Segmentation.
Medical Physics(2020)
关键词
data augmentation,deep learning,image warping,medical image segmentation
AI 理解论文
溯源树
样例
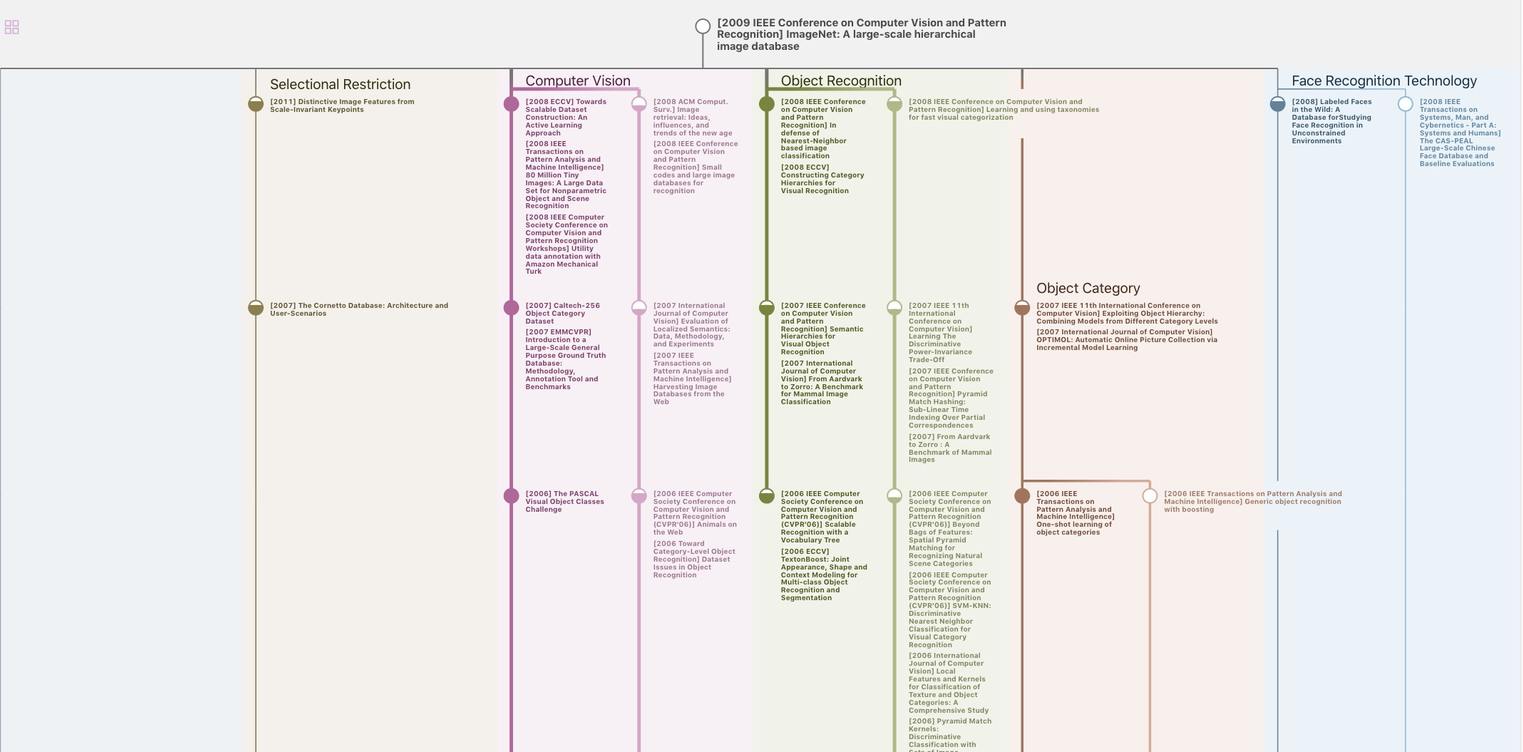
生成溯源树,研究论文发展脉络
Chat Paper
正在生成论文摘要
Medical Physics(2020)