Explainable-by-design Semi-Supervised Representation Learning for COVID-19 Diagnosis from CT Imaging
Abel Díaz Berenguer,Hichem Sahli,Boris Joukovsky,Maryna Kvasnytsia,Ine Dirks,Mitchel Alioscha-Perez,Nikos Deligiannis,Panagiotis Gonidakis,Sebastián Amador Sánchez,Redona Brahimetaj,Evgenia Papavasileiou, Jonathan Cheung-Wai Chana, Fei Li,Shangzhen Song,Yixin Yang,Sofie Tilborghs,Siri Willems,Tom Eelbode,Jeroen Bertels,Dirk Vandermeulen,Frederik Maes,Paul Suetens,Lucas Fidon,Tom Vercauteren,David Robben,Arne Brys,Dirk Smeets,Bart Ilsen,Nico Buls, Nina Watté,Johan de Mey,Annemiek Snoeckx,Paul M. Parizel,Julien Guiot,Louis Deprez,Paul Meunier,Stefaan Gryspeerdt,Kristof De Smet,Bart Jansen,Jef Vandemeulebroucke Zenodo (CERN European Organization for Nuclear Research)(2022)
AI Read Science
Must-Reading Tree
Example
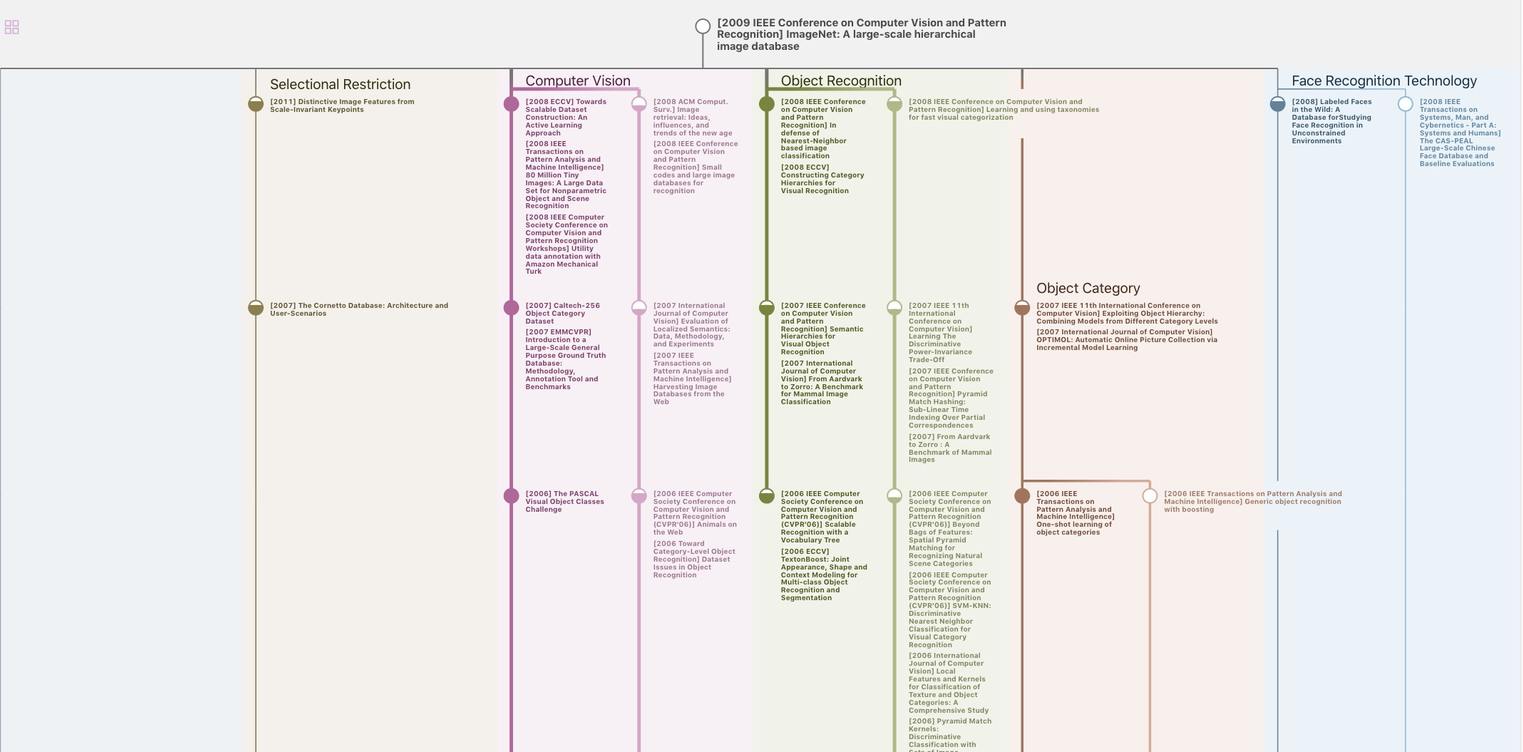
Generate MRT to find the research sequence of this paper