OGNet: Towards a Global Oil and Gas Infrastructure Database Using Deep Learning on Remotely Sensed Imagery
CoRR(2020)
Key words
Emissions,Oil Spill Detection,Oil Spill Modeling,Deep Learning,Remote Sensing
AI Read Science
Must-Reading Tree
Example
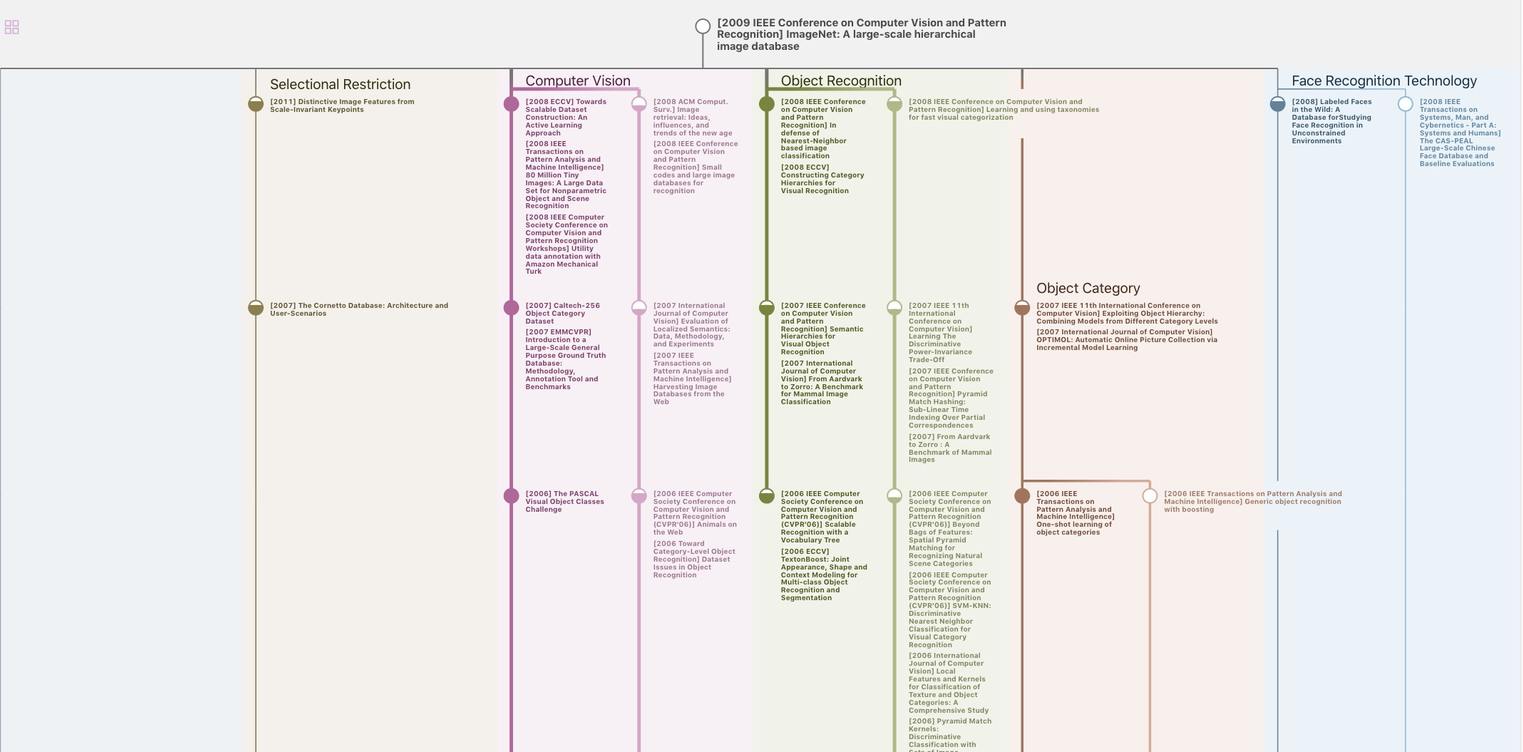
Generate MRT to find the research sequence of this paper
Chat Paper
Summary is being generated by the instructions you defined