Selecting Optimal Completion to Partial Matrix via Self Validation
IEEE Signal Processing Letters(2020)
摘要
In many applications such as film recommendation, one often encounters the problem of estimating the unseen entries in a partially observed matrix, formally known as matrix completion. Over the past several decades, lots of effective methods have been established in the literature, and each method may contain several hyper-parameters. For a partial matrix, one can use those methods with certain parametric settings to obtain a large number of completions. Now, a critical question is, how to select the optimal completion from a number of candidates ? This question is indeed a hard to answer, because in practice the true values of the missing entries are unknown. Thus far, the only approach for dealing with the issue is through data-validation , which is to first split the observations into two subsets, a training set and a validation set, and then choose the model that performs best on the validation set as the winner to …
更多查看译文
关键词
Matrix completion, identifiability measure, self-validation, isomeric condition
AI 理解论文
溯源树
样例
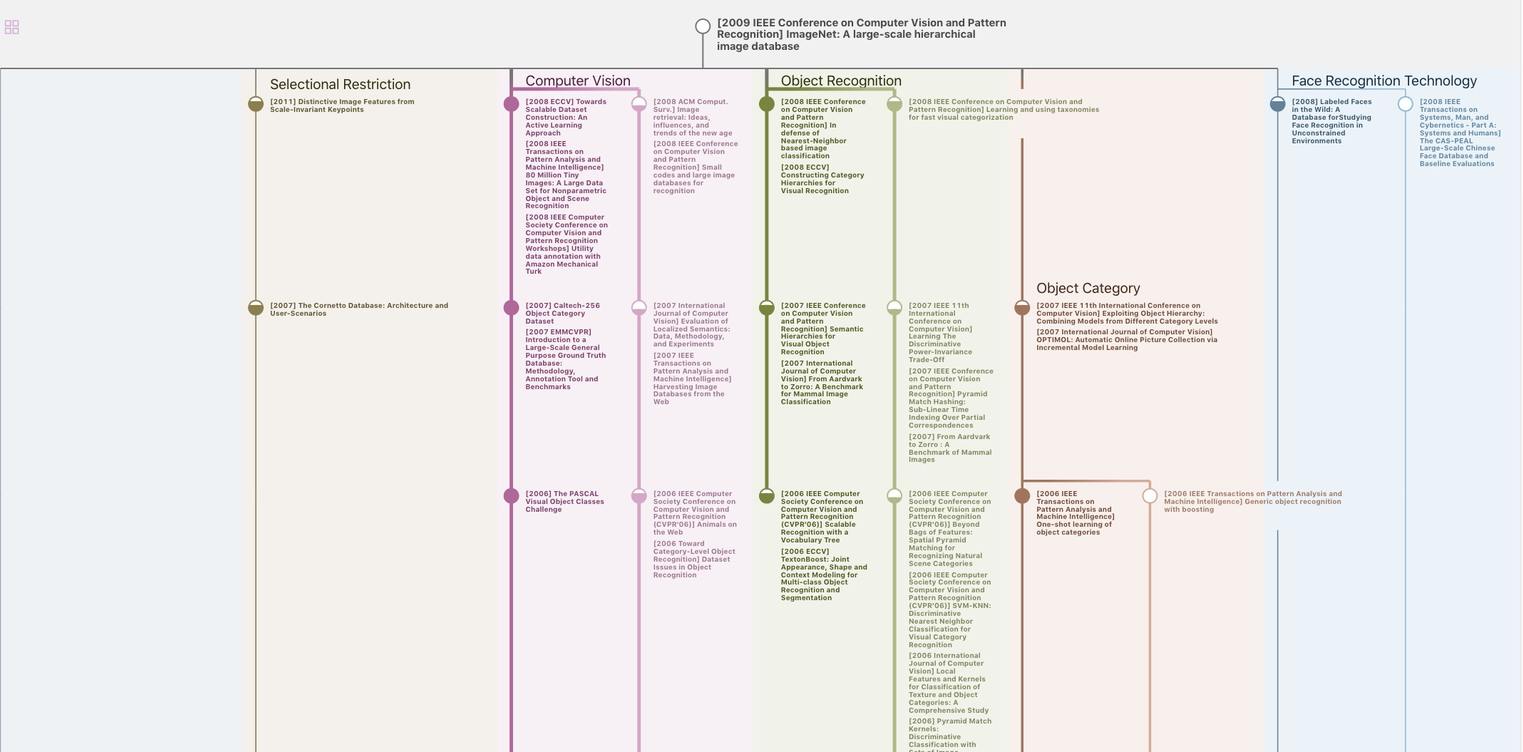
生成溯源树,研究论文发展脉络
Chat Paper
正在生成论文摘要