Scalable Gaussian Processes, with Guarantees: Kernel Approximations and Deep Feature Extraction
arXiv preprint arXiv:2004.01584(2020)
AI Read Science
Must-Reading Tree
Example
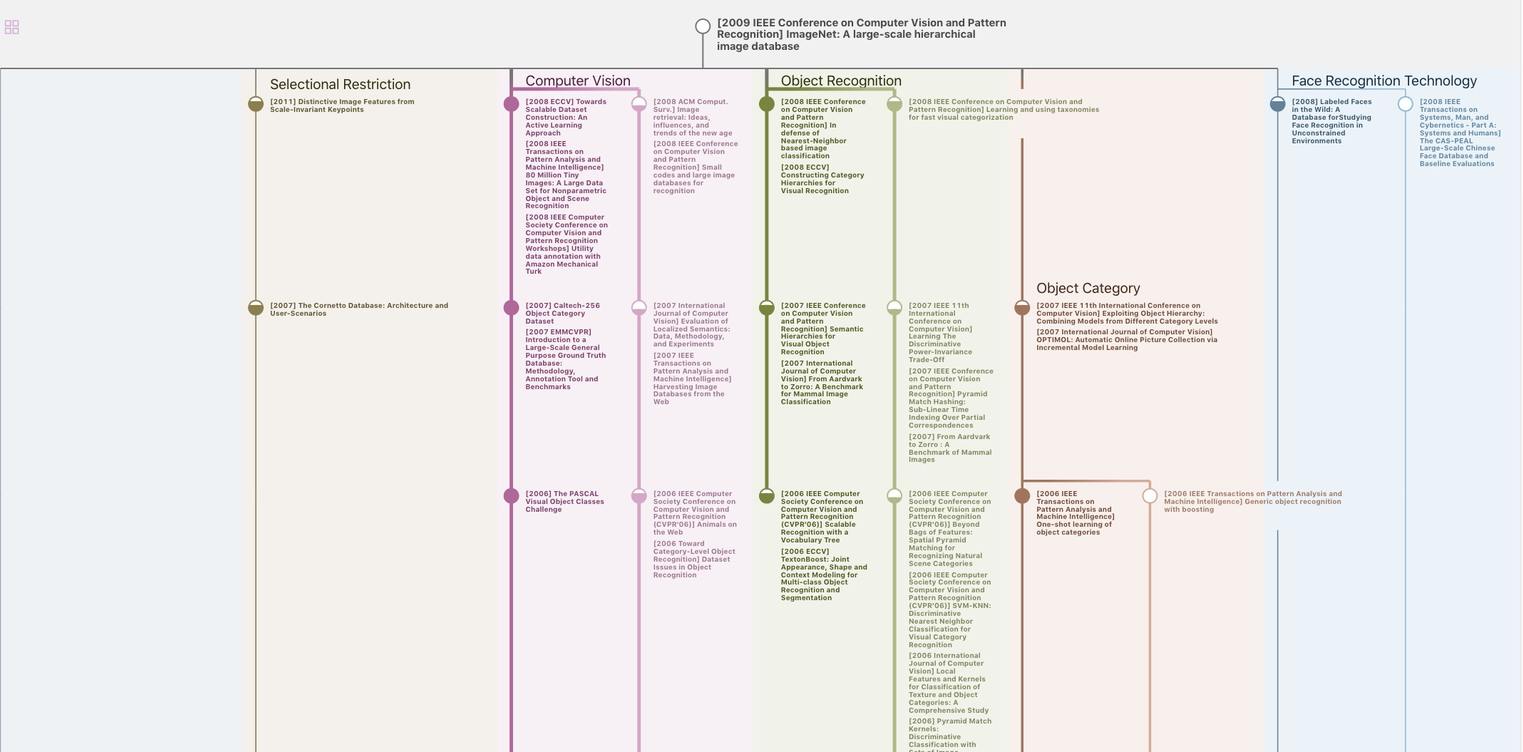
Generate MRT to find the research sequence of this paper
Chat Paper
Summary is being generated by the instructions you defined