Integrating Categorical Semantics into Unsupervised Domain Translation.
ICLR 2021(2021)
Key words
Unsupervised Domain Translation,Unsupervised Learning,Image-to-Image Translation,Deep Learning,Representation Learning
AI Read Science
Must-Reading Tree
Example
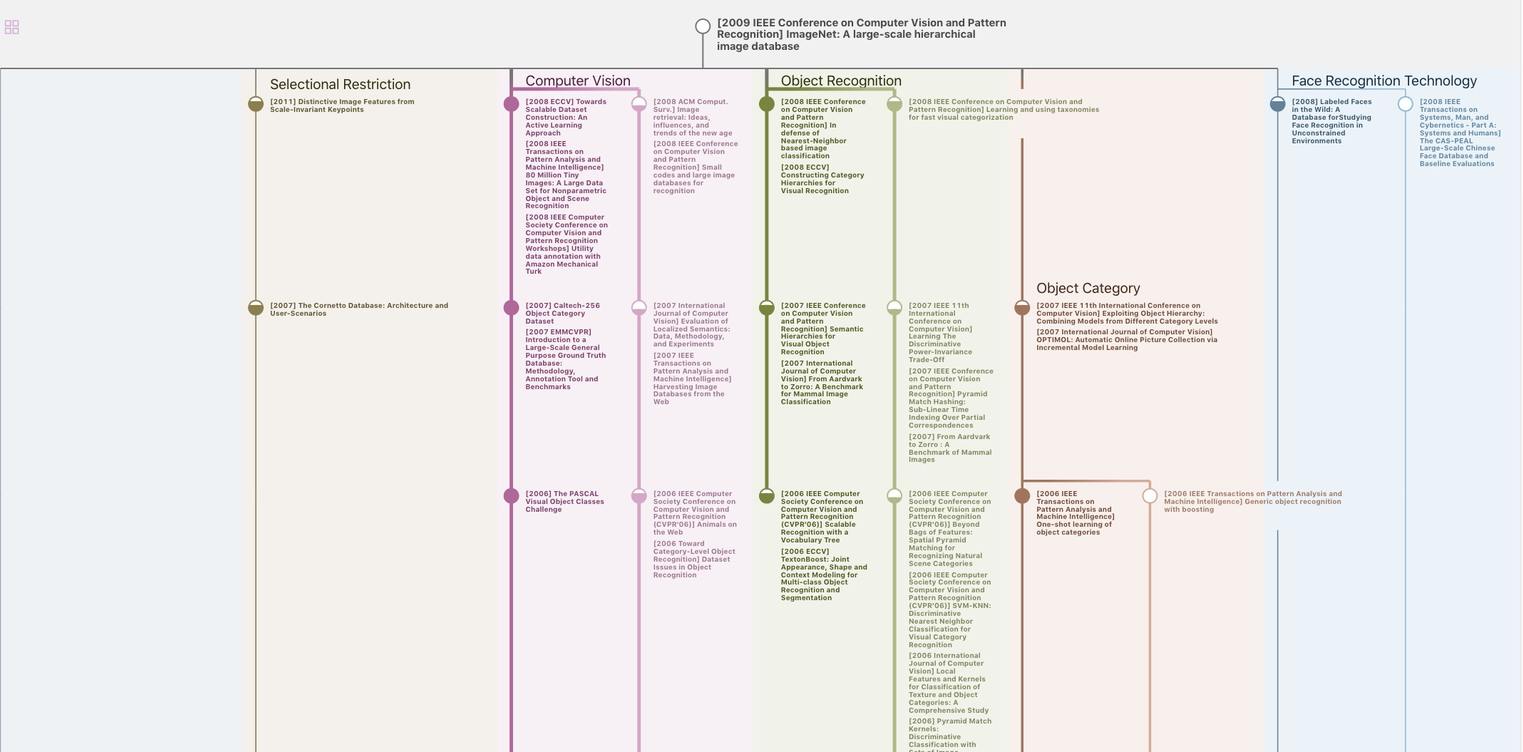
Generate MRT to find the research sequence of this paper
Chat Paper
Summary is being generated by the instructions you defined