Nonlinear Extensions of Reconstruction ICA
semanticscholar(2011)
摘要
In a recent paper [1] it was observed that unsupervised feature learning with overcomplete features could be achieved using linear autoencoders (named Reconstruction Independent Component Analysis). This algorithm has been shown to outperform other well-known algorithms by penalizing the lack of diversity (or orthogonality) amongst features. In our project, we wish to extend and improve this algorithm to include other non-linearities. In this project we have considered three unsupervised learning algorithms: (a) Sparse Autoencoder (b) Reconstruction ICA (RICA), a linear autoencoder proposed in [1], and (c) Nonlinear RICA, a proposed extension of RICA for capturing nonlinearities in feature detection. Our research indicates that exploring non-linear extensions of RICA holds good promise; preliminary results with hyperbolic tangent function on the MNIST dataset showed impressive accuracy (comparable with sparse autoencoders), robustness, and required a fraction of the computational effort.
更多查看译文
AI 理解论文
溯源树
样例
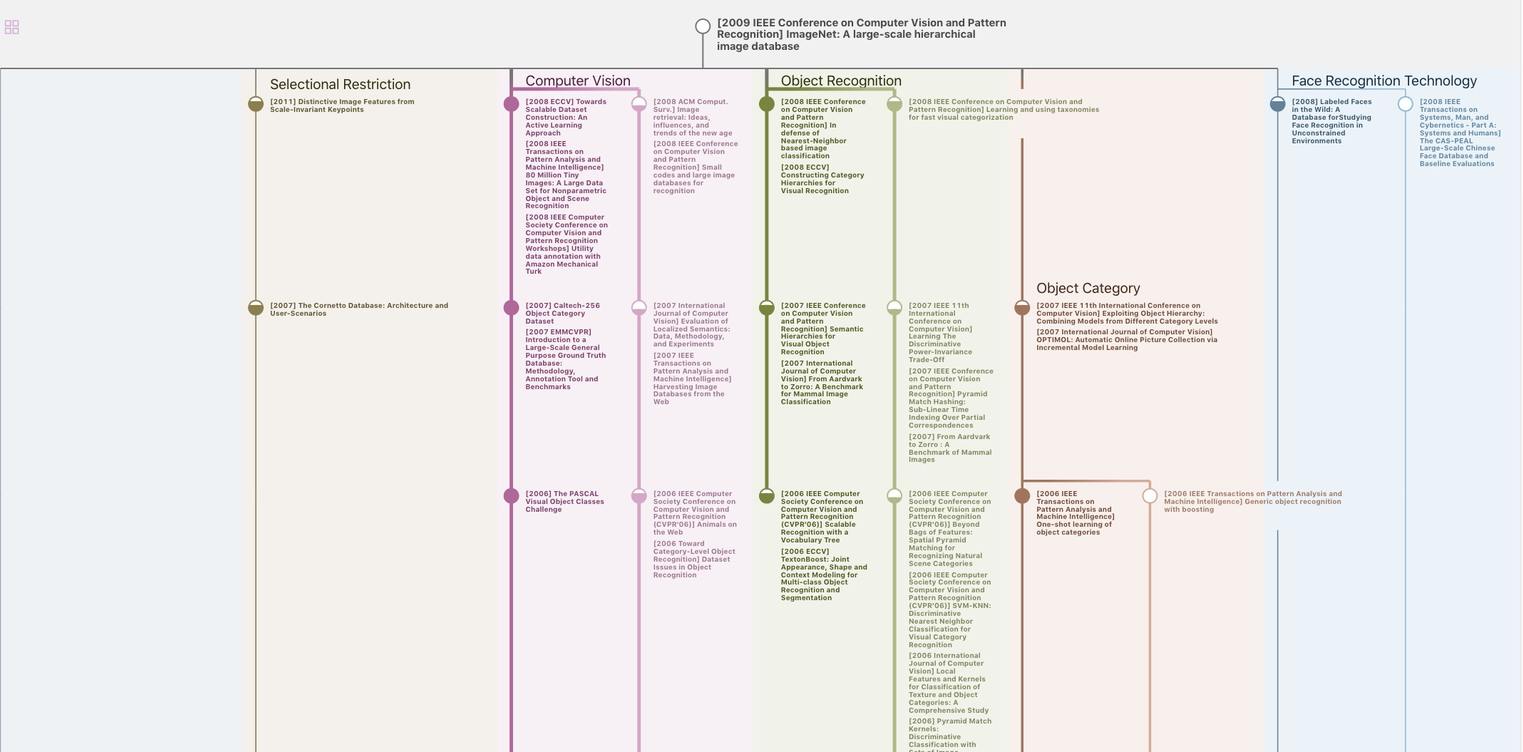
生成溯源树,研究论文发展脉络
Chat Paper
正在生成论文摘要