Domain-Adaptive Neural Automated Essay Scoring
Annual International ACM SIGIR Conference on Research and Development in Information Retrieval(2020)
Key words
Automated essay scoring,natural language processing,domain adaptation,self-supervised learning
AI Read Science
Must-Reading Tree
Example
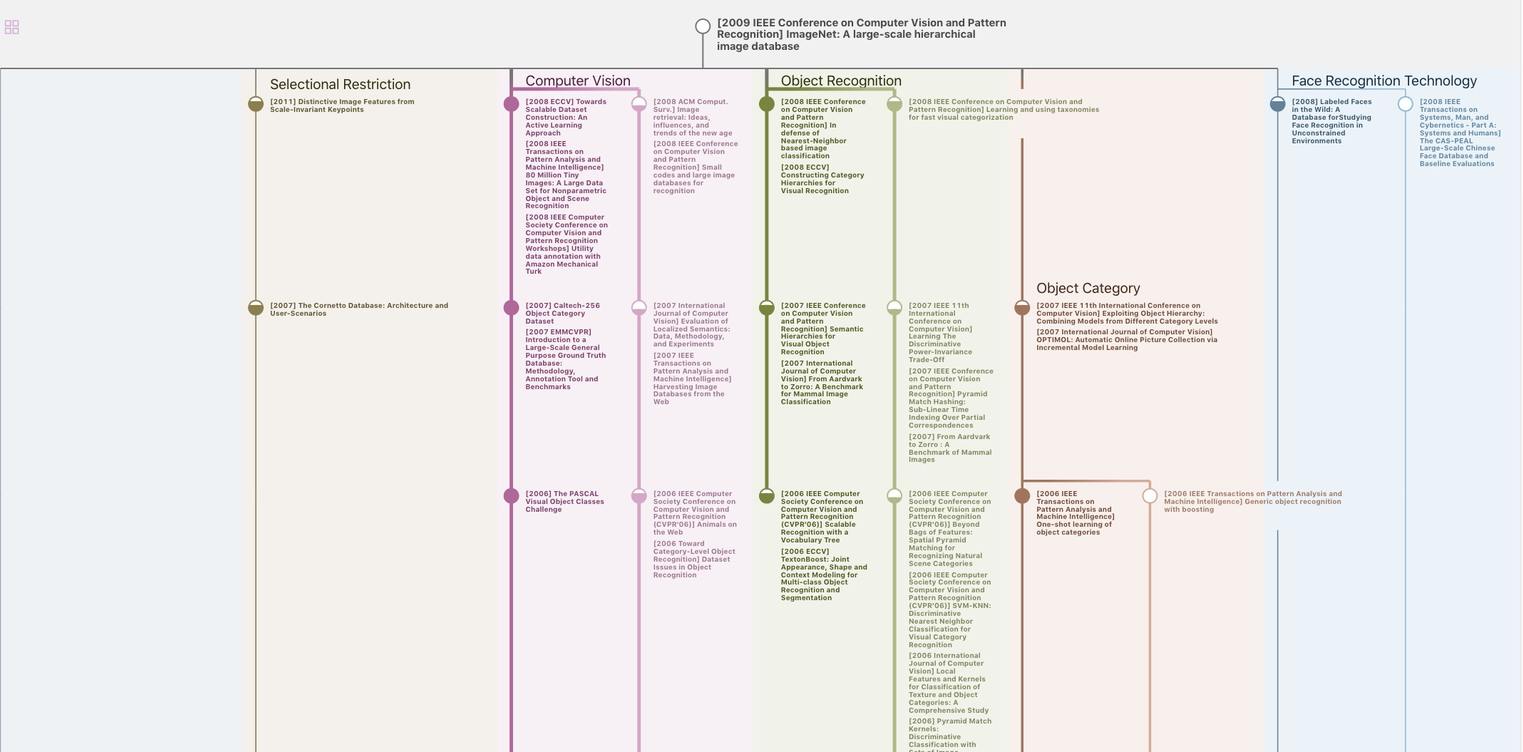
Generate MRT to find the research sequence of this paper
Chat Paper
Summary is being generated by the instructions you defined