Anomaly Detection in Mobile Networks.
2020 IEEE Wireless Communications and Networking Conference Workshops (WCNCW)(2020)
Key words
Time Series Analysis,anomaly detection,Self-organizing networks
AI Read Science
Must-Reading Tree
Example
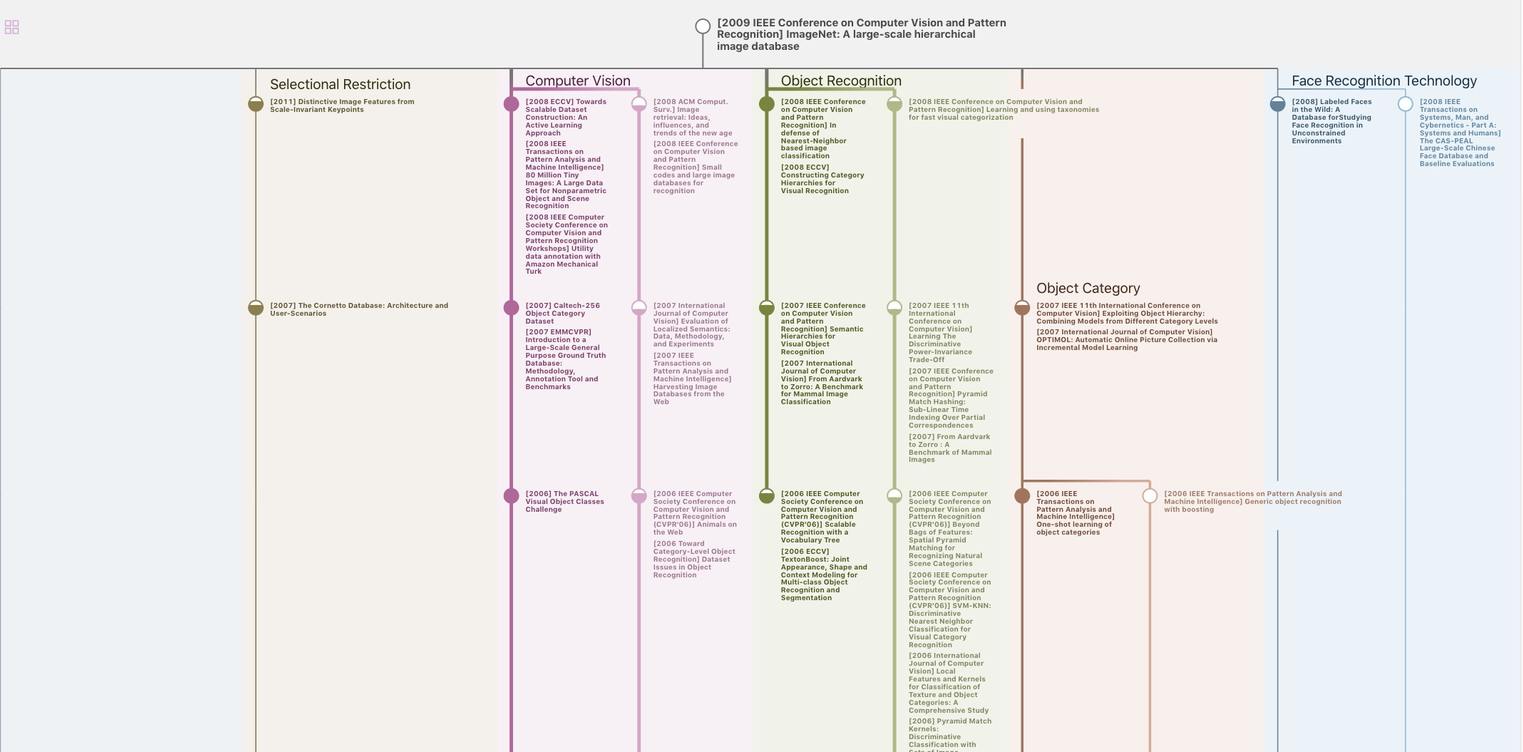
Generate MRT to find the research sequence of this paper
Chat Paper
Summary is being generated by the instructions you defined