HMCTS-OP: Hierarchical MCTS Based Online Planning in the Asymmetric Adversarial Environment.
SYMMETRY-BASEL(2020)
摘要
The Monte Carlo Tree Search (MCTS) has demonstrated excellent performance in solving many planning problems. However, the state space and the branching factors are huge, and the planning horizon is long in many practical applications, especially in the adversarial environment. It is computationally expensive to cover a sufficient number of rewarded states that are far away from the root in the flat non-hierarchical MCTS. Therefore, the flat non-hierarchical MCTS is inefficient for dealing with planning problems with a long planning horizon, huge state space, and branching factors. In this work, we propose a novel hierarchical MCTS-based online planning method named the HMCTS-OP to tackle this issue. The HMCTS-OP integrates the MAXQ-based task hierarchies and the hierarchical MCTS algorithms into the online planning framework. Specifically, the MAXQ-based task hierarchies reduce the search space and guide the search process. Therefore, the computational complexity is significantly reduced. Moreover, the reduction in the computational complexity enables the MCTS to perform a deeper search to find better action in a limited time. We evaluate the performance of the HMCTS-OP in the domain of online planning in the asymmetric adversarial environment. The experiment results show that the HMCTS-OP outperforms other online planning methods in this domain.
更多查看译文
关键词
HMCTS,online planning,MAXQ,asymmetric adversarial environment
AI 理解论文
溯源树
样例
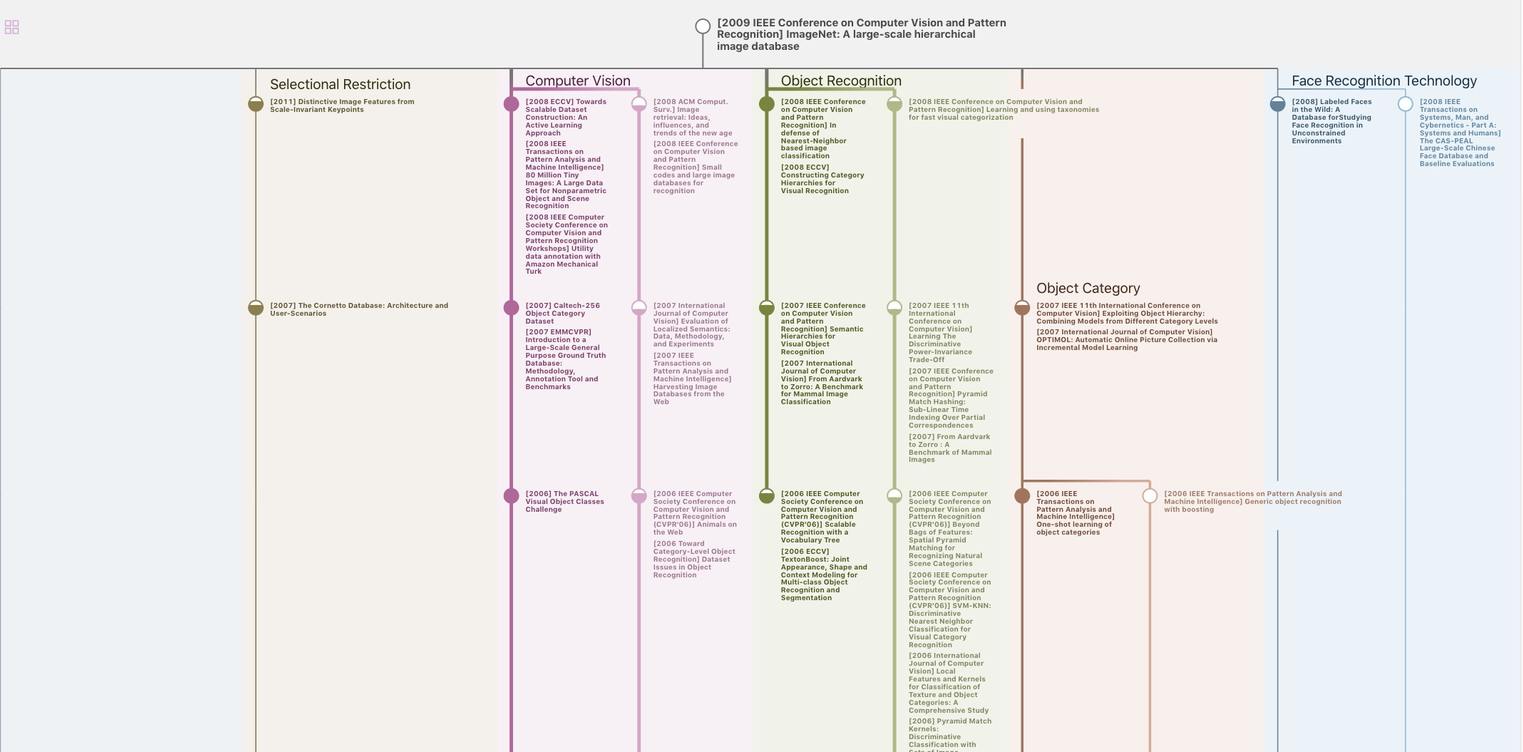
生成溯源树,研究论文发展脉络
Chat Paper
正在生成论文摘要