Rapid Data-Driven Model Reduction of Nonlinear Dynamical Systems Including Chemical Reaction Networks Using ℓ1-Regularization.
Chaos An Interdisciplinary Journal of Nonlinear Science(2020)
AI Read Science
Must-Reading Tree
Example
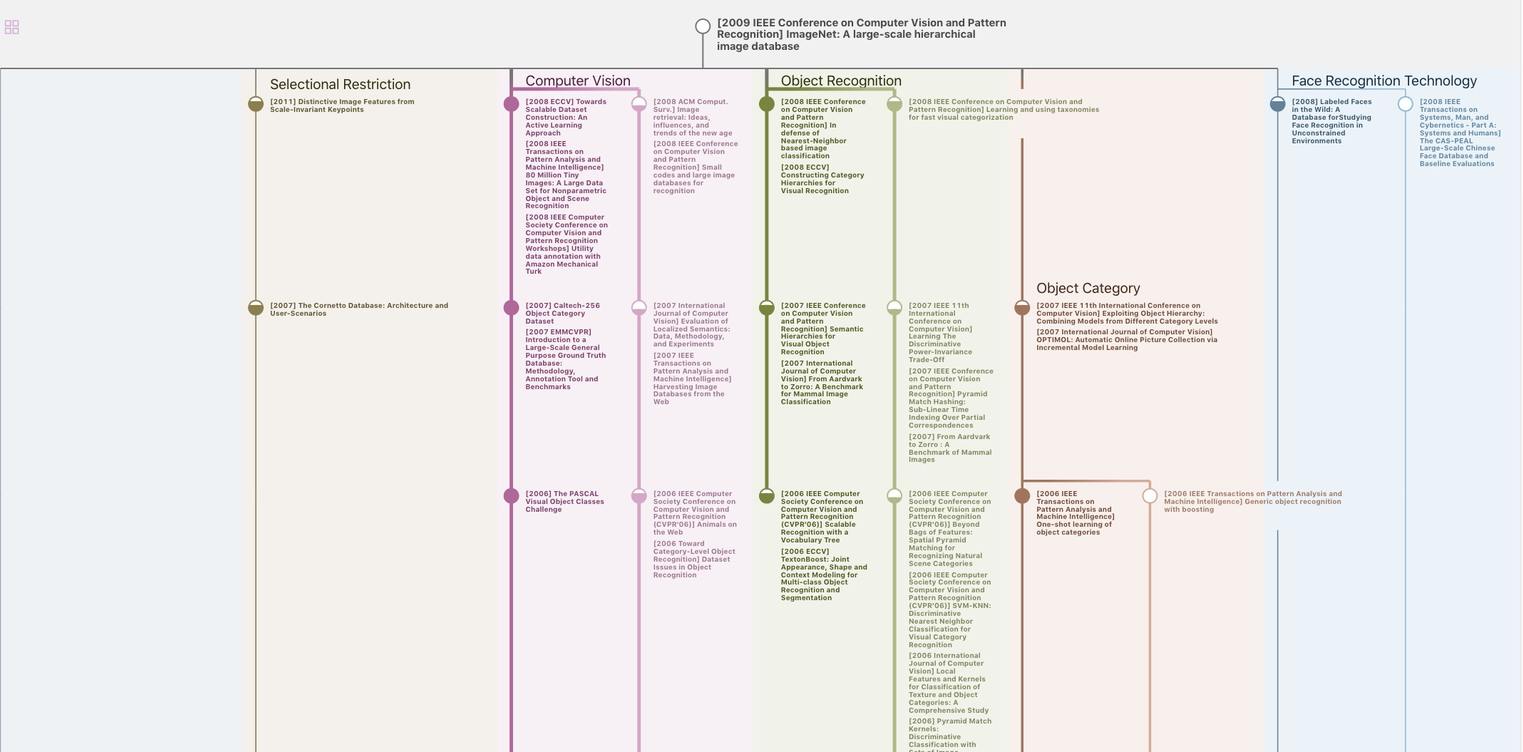
Generate MRT to find the research sequence of this paper
Chat Paper
Summary is being generated by the instructions you defined