User Mobility Synthesis Based on Generative Adversarial Networks: A Survey
2020 22ND INTERNATIONAL CONFERENCE ON ADVANCED COMMUNICATION TECHNOLOGY (ICACT) DIGITAL SECURITY GLOBAL AGENDA FOR SAFE SOCIETY(2020)
关键词
user mobility,mobility synthesis,mobility generation,mobility trace synthesis,mobility trace generation,generative adversarial networks,GAN
AI 理解论文
溯源树
样例
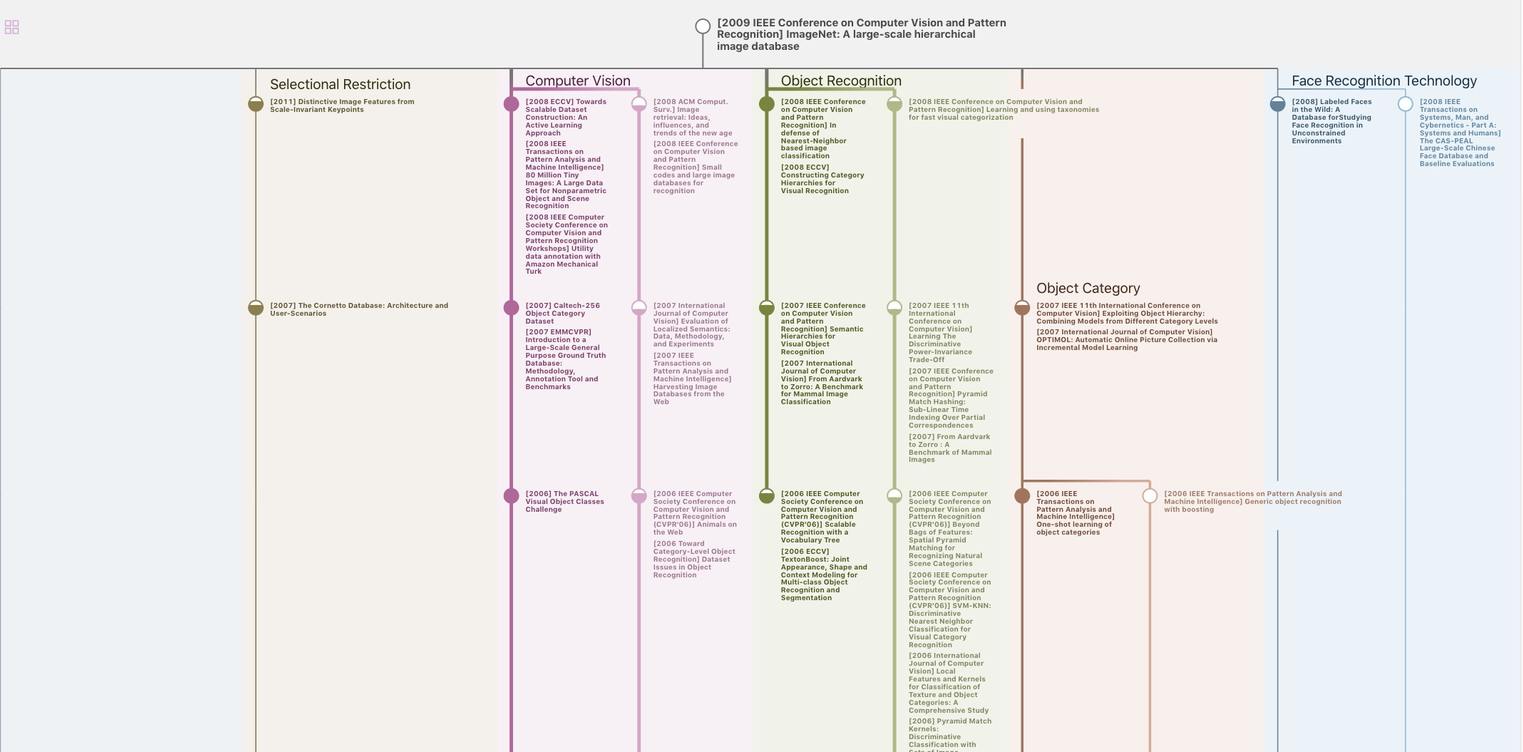
生成溯源树,研究论文发展脉络
Chat Paper
正在生成论文摘要