Bayesian Integrative Modeling of Genome-Scale Metabolic and Regulatory Networks
INFORMATICS-BASEL(2020)
Univ Nantes
Abstract
The integration of high-throughput data to build predictive computational models of cellular metabolism is a major challenge of systems biology. These models are needed to predict cellular responses to genetic and environmental perturbations. Typically, this response involves both metabolic regulations related to the kinetic properties of enzymes and a genetic regulation affecting their concentrations. Thus, the integration of the transcriptional regulatory information is required to improve the accuracy and predictive ability of metabolic models. Integrative modeling is of primary importance to guide the search for various applications such as discovering novel potential drug targets to develop efficient therapeutic strategies for various diseases. In this paper, we propose an integrative predictive model based on techniques combining semantic web, probabilistic modeling, and constraint-based modeling methods. We applied our approach to human cancer metabolism to predict in silico the growth response of specific cancer cells under approved drug effects. Our method has proven successful in predicting the biomass rates of human liver cancer cells under drug-induced transcriptional perturbations.
MoreTranslated text
Key words
metabolic networks,transcriptional regulatory networks,probabilistic model,integrative modeling,constraint-based modeling
PDF
View via Publisher
AI Read Science
Must-Reading Tree
Example
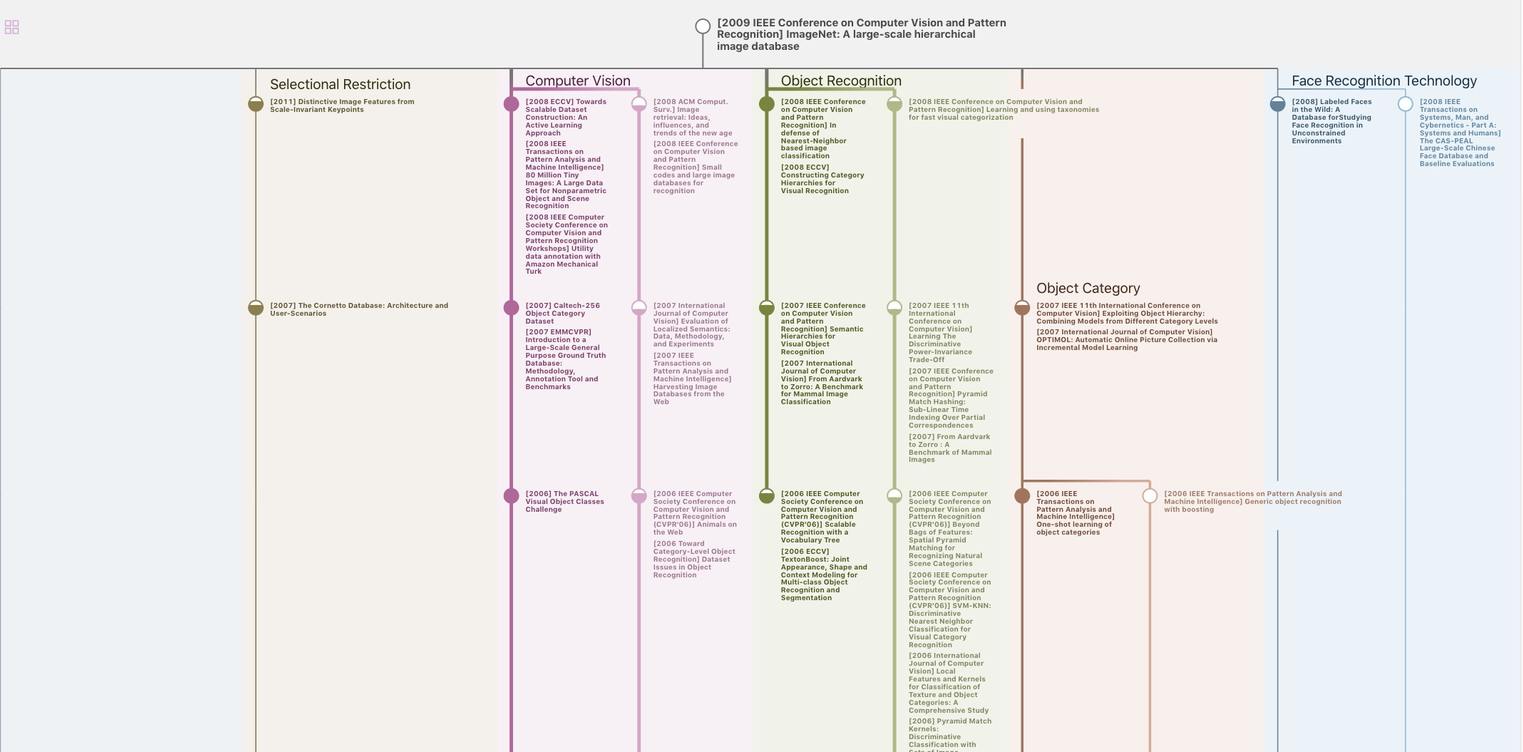
Generate MRT to find the research sequence of this paper
Data Disclaimer
The page data are from open Internet sources, cooperative publishers and automatic analysis results through AI technology. We do not make any commitments and guarantees for the validity, accuracy, correctness, reliability, completeness and timeliness of the page data. If you have any questions, please contact us by email: report@aminer.cn
Chat Paper
Summary is being generated by the instructions you defined