There is Strength in Numbers: Avoiding the Hypothesis-Only Bias in Natural Language Inference via Ensemble Adversarial Training
arxiv(2020)
摘要
Natural Language Inference (NLI) datasets contain annotation artefacts resulting in spurious correlations between the natural language utterances and their respective entailment classes. These artefacts are exploited by neural networks even when only considering the hypothesis and ignoring the premise, leading to unwanted biases. Previous work proposed tackling this problem via adversarial training, but this leads to learned sentence representations that still suffer from the same biases. As a solution, we propose using an ensemble of adversaries during the training, encouraging the model to jointly decrease the accuracy of these different adversaries while fitting the data. We show that using an ensemble of adversaries can prevent the bias from being relearned after the model training is completed, further improving how well the model generalises to different NLI datasets. In particular, these models outperformed previous approaches when tested on 12 different NLI datasets not used in the model training. Finally, the optimal number of adversarial classifiers depends on the dimensionality of the sentence representations, with larger dimensional representations benefiting when trained with a greater number of adversaries.
更多查看译文
关键词
ensemble adversarial training,natural language inference,bias,hypothesis-only
AI 理解论文
溯源树
样例
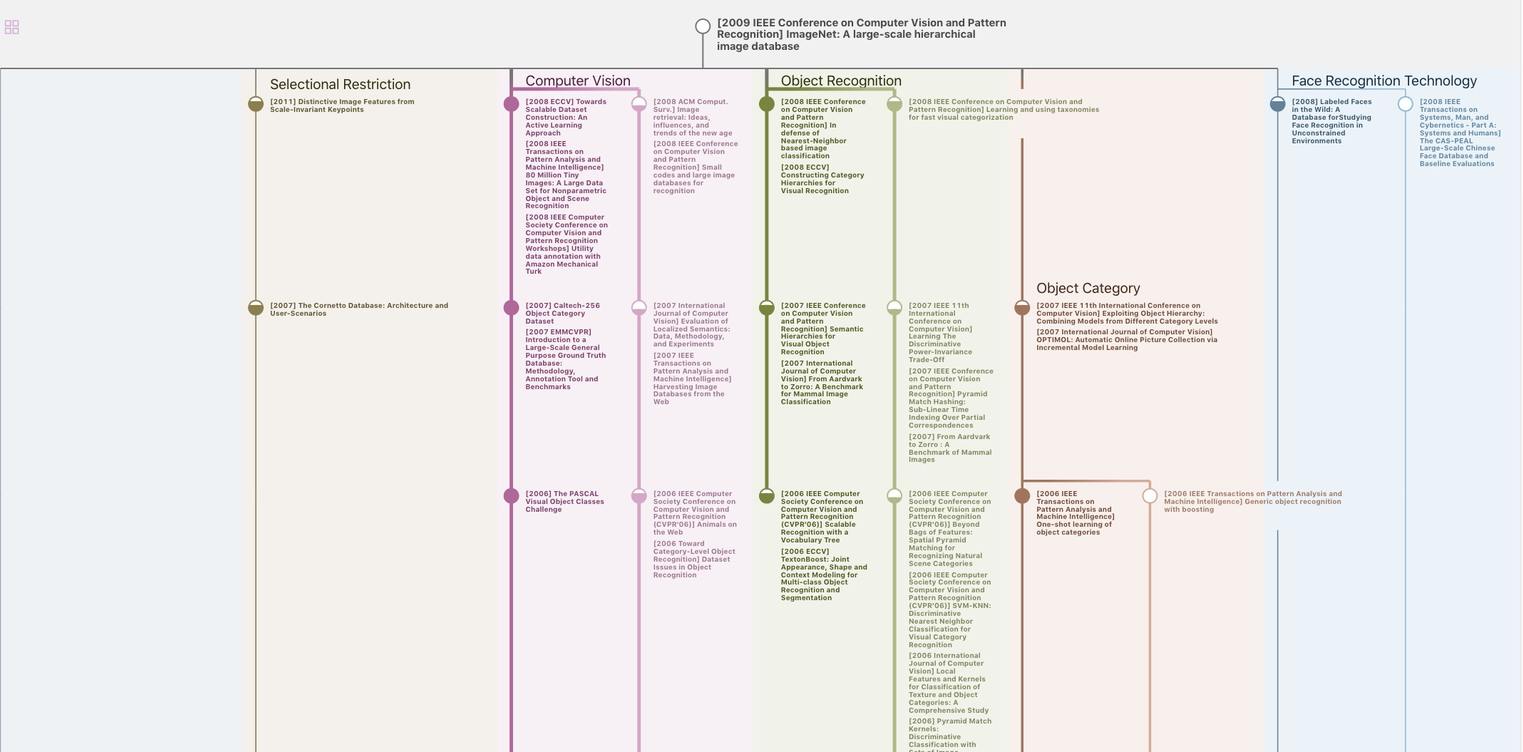
生成溯源树,研究论文发展脉络
Chat Paper
正在生成论文摘要