Query-Biased Self-Attentive Network for Query-Focused Video Summarization.
IEEE Transactions on Image Processing(2020)
关键词
Task analysis,Semantics,Visualization,Computational modeling,Generators,Benchmark testing,Instruments,Video summarization,vision and language,self-attention mechanism
AI 理解论文
溯源树
样例
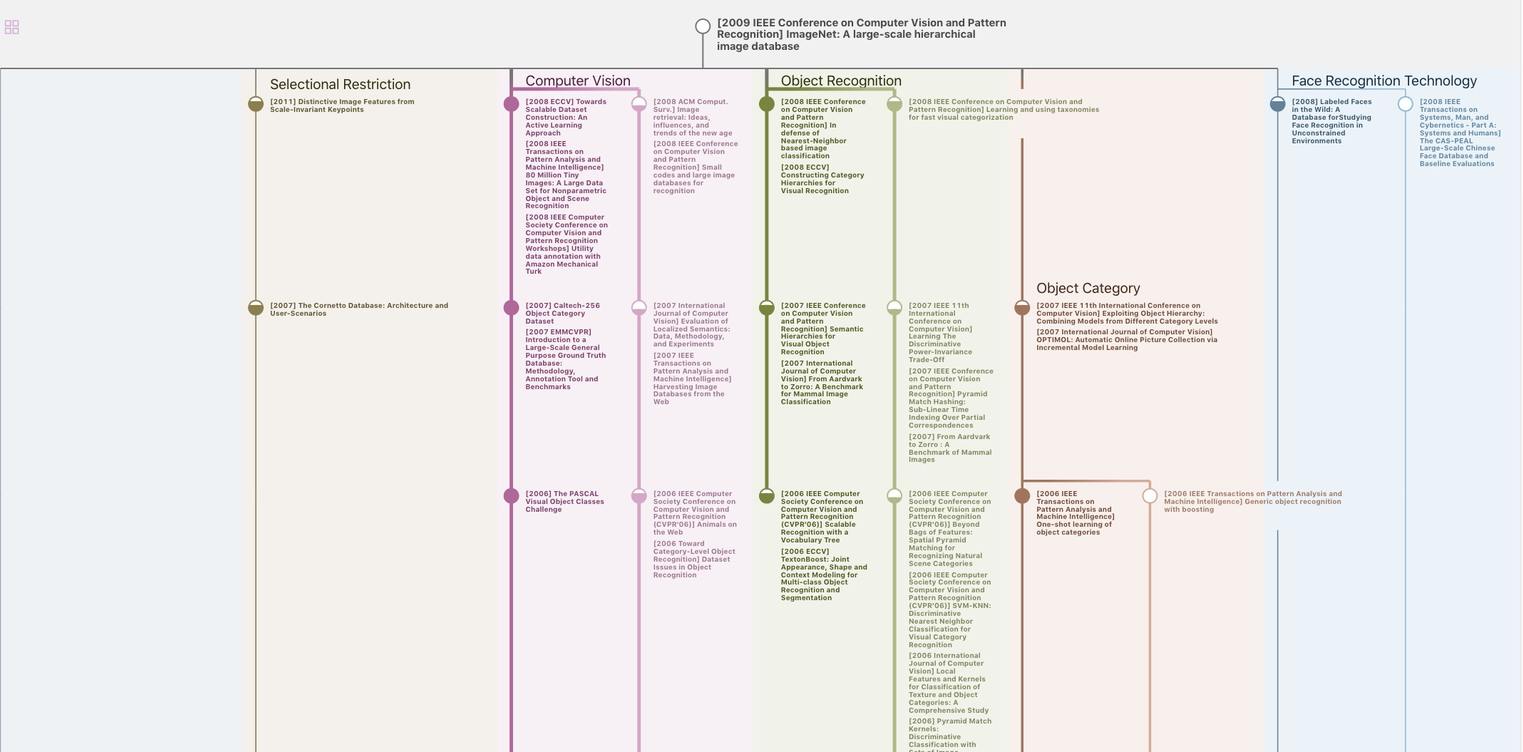
生成溯源树,研究论文发展脉络
Chat Paper
正在生成论文摘要