Hyper-local, Efficient Extreme Heat Projection and Analysis Using Machine Learning to Augment a Hybrid Dynamical-Statistical Downscaling Technique
Urban Climate(2020)
关键词
Climate downscaling,Extreme heat
AI 理解论文
溯源树
样例
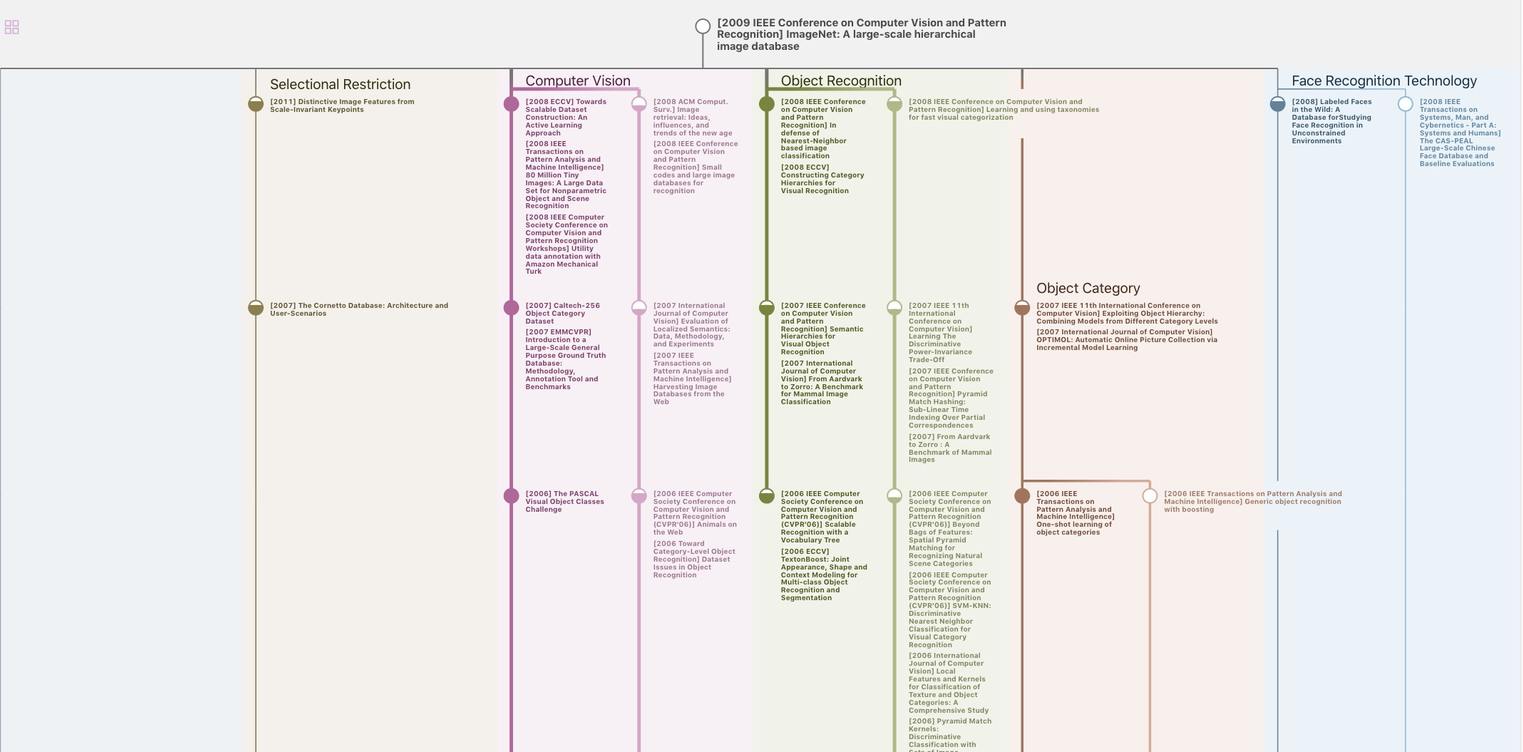
生成溯源树,研究论文发展脉络
Chat Paper
正在生成论文摘要