Ecological Prediction at Macroscales Using Big Data: Does Sampling Design Matter?
ECOLOGICAL APPLICATIONS(2020)
Key words
data-intensive ecology,ecological context,extrapolation,interpolation,lakes,macroscale,monitoring,prediction,sampling,sampling design
AI Read Science
Must-Reading Tree
Example
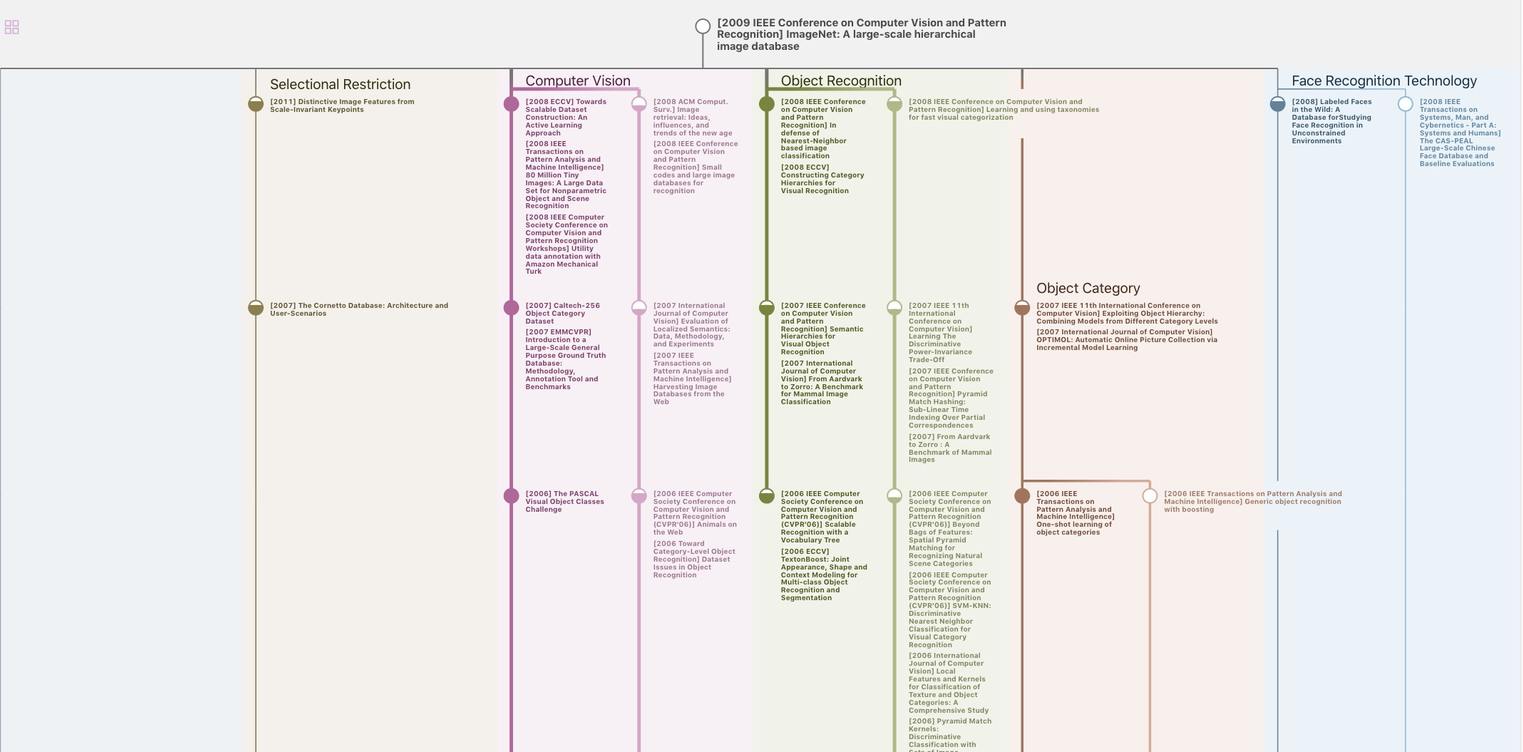
Generate MRT to find the research sequence of this paper
Chat Paper
Summary is being generated by the instructions you defined