Matrix-variate Variational Auto-Encoder with Applications to Image Process
Journal of Visual Communication and Image Representation(2020)
关键词
Variational autoencoder,Matrix Gaussian distribution,Variational inference,Face completion,Image denoising
AI 理解论文
溯源树
样例
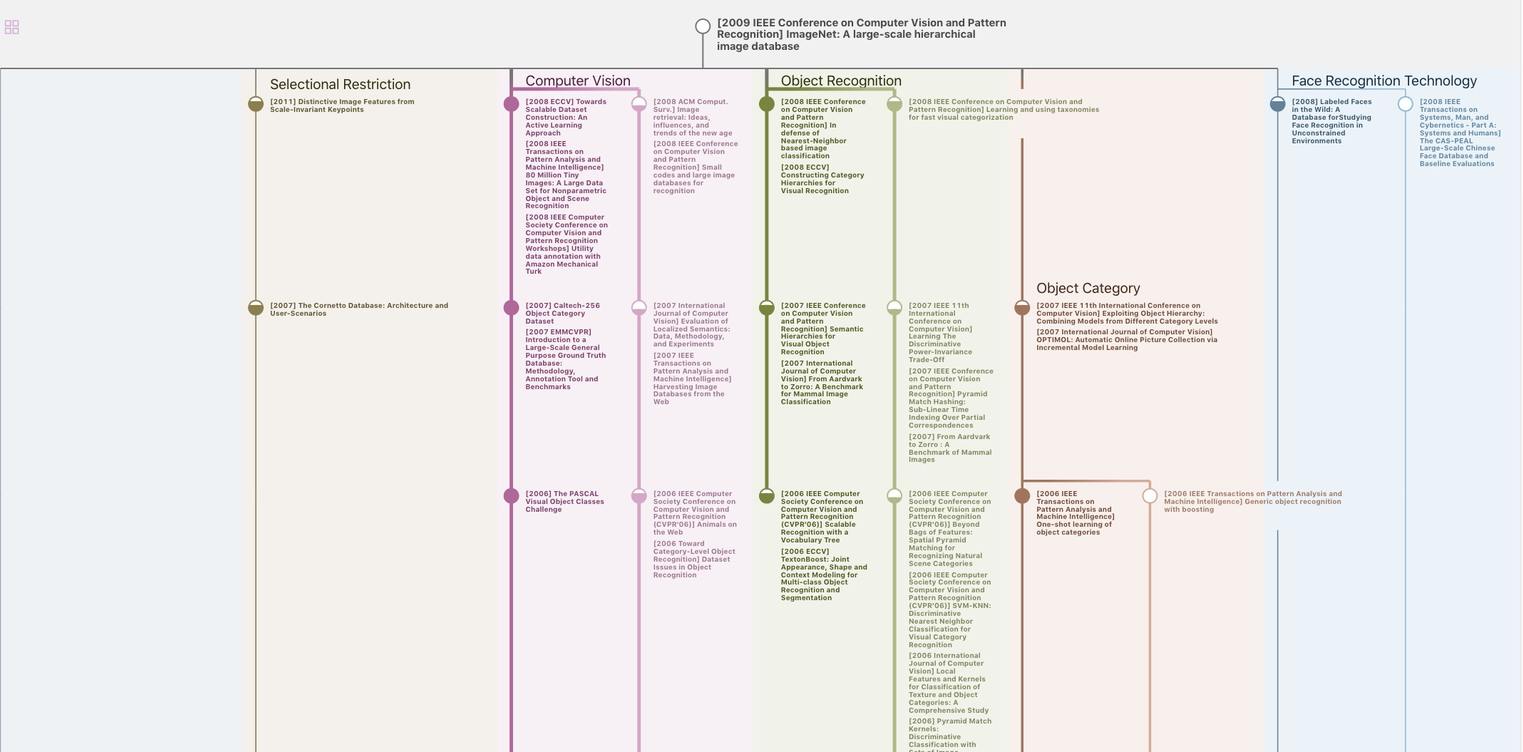
生成溯源树,研究论文发展脉络
Chat Paper
正在生成论文摘要