Hyperspectral Image Classification Based on 3D Asymmetric Inception Network with Data Fusion Transfer Learning
CoRR(2020)
Key words
hyperspectral image classification,convolutional neural network,light-weight network,3D asymmetric inception network,transfer learning
AI Read Science
Must-Reading Tree
Example
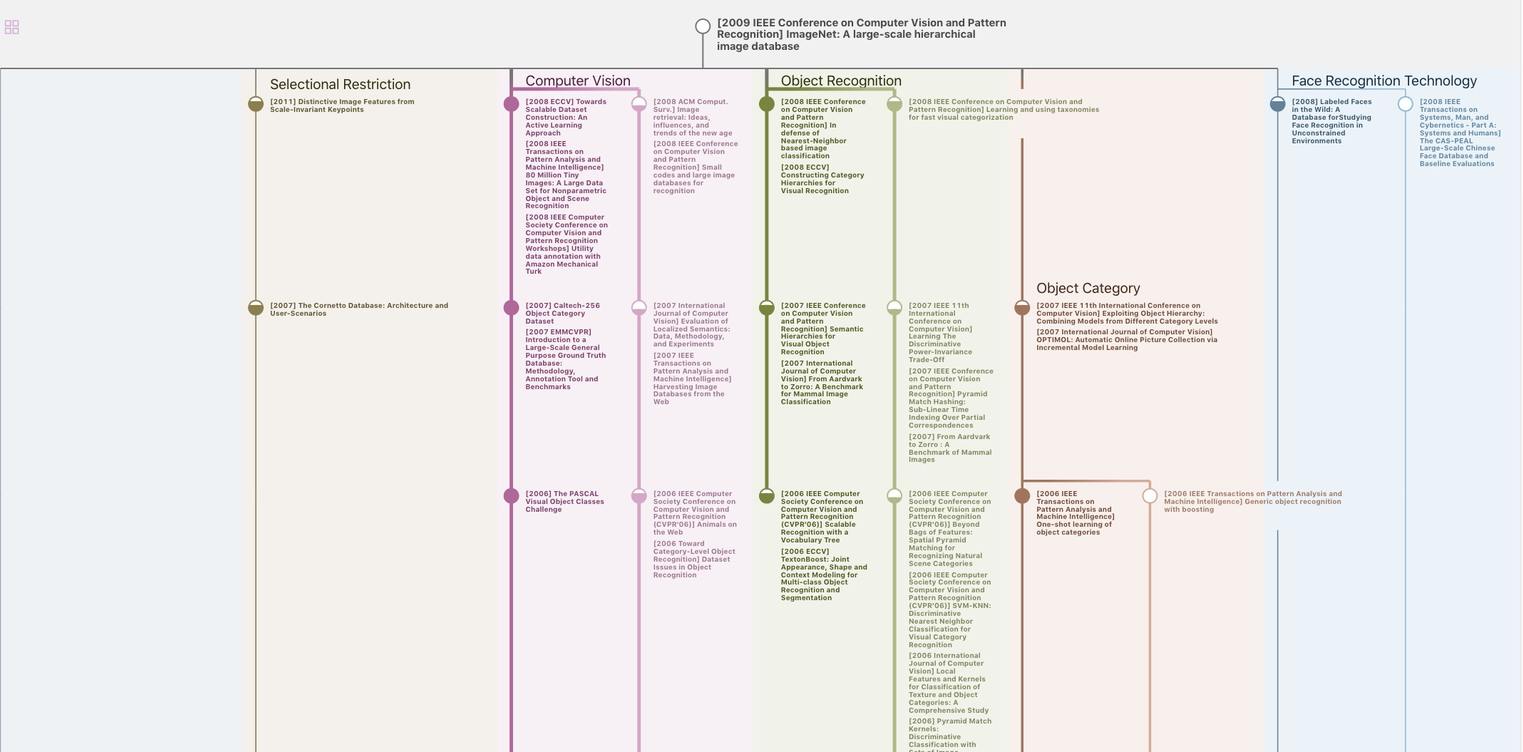
Generate MRT to find the research sequence of this paper
Chat Paper
Summary is being generated by the instructions you defined