Causally Correct Partial Models for Reinforcement Learning
ICLR 2020(2020)
Key words
causality,model-based reinforcement learning
AI Read Science
Must-Reading Tree
Example
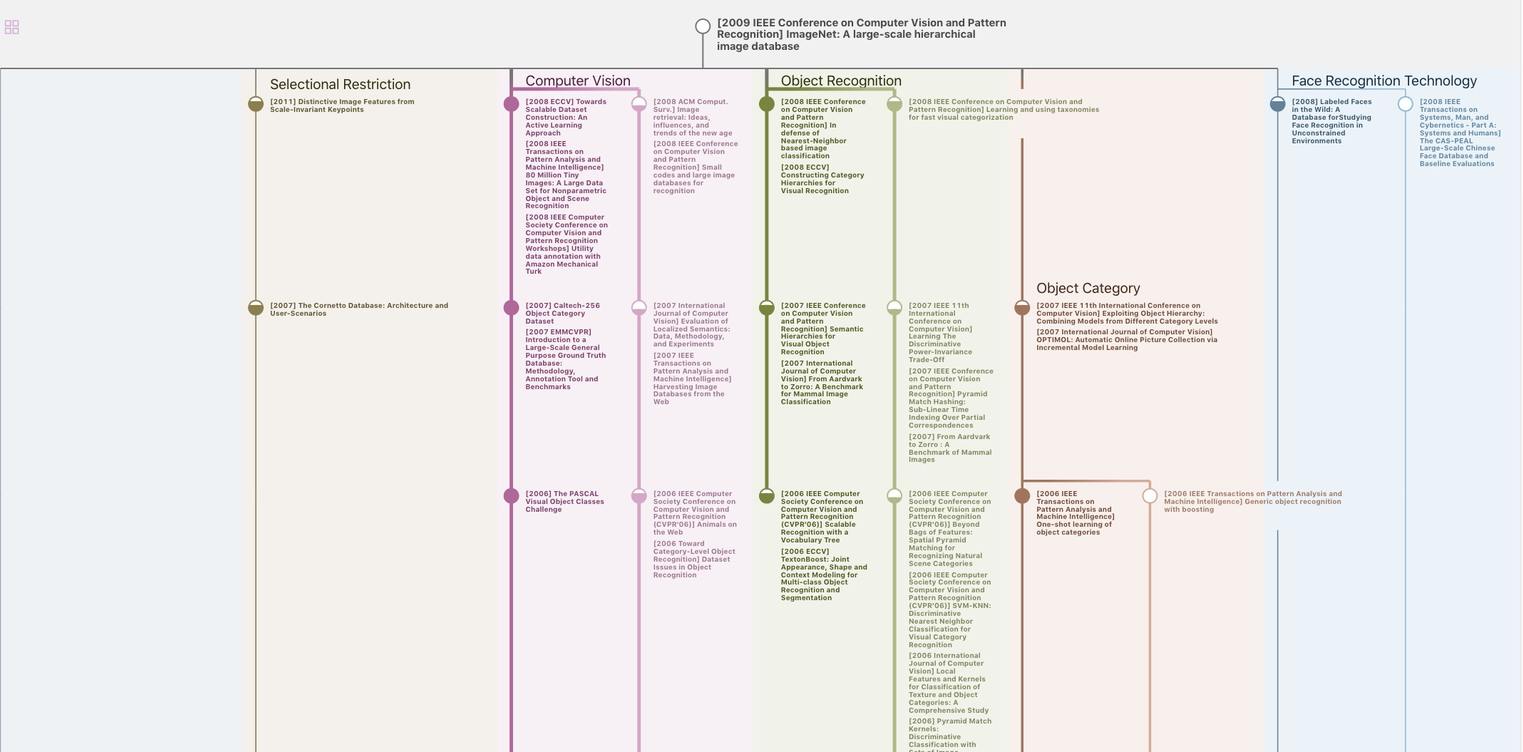
Generate MRT to find the research sequence of this paper
Chat Paper
Summary is being generated by the instructions you defined