Automatic Scoring of Diabetic Foot Ulcers Through Deep CNN Based Feature Extraction with Low Rank Matrix Factorization
2019 IEEE 19th International Conference on Bioinformatics and Bioengineering (BIBE)(2019)
Key words
Diabetic Foot Ulcer (DFU),Convolutional Neural Network (CNN),Transfer Learning,Global Average Pooling (GAP),Singular Value Decomposition (SVD),Classification,Artificial Neural Network (ANN)
AI Read Science
Must-Reading Tree
Example
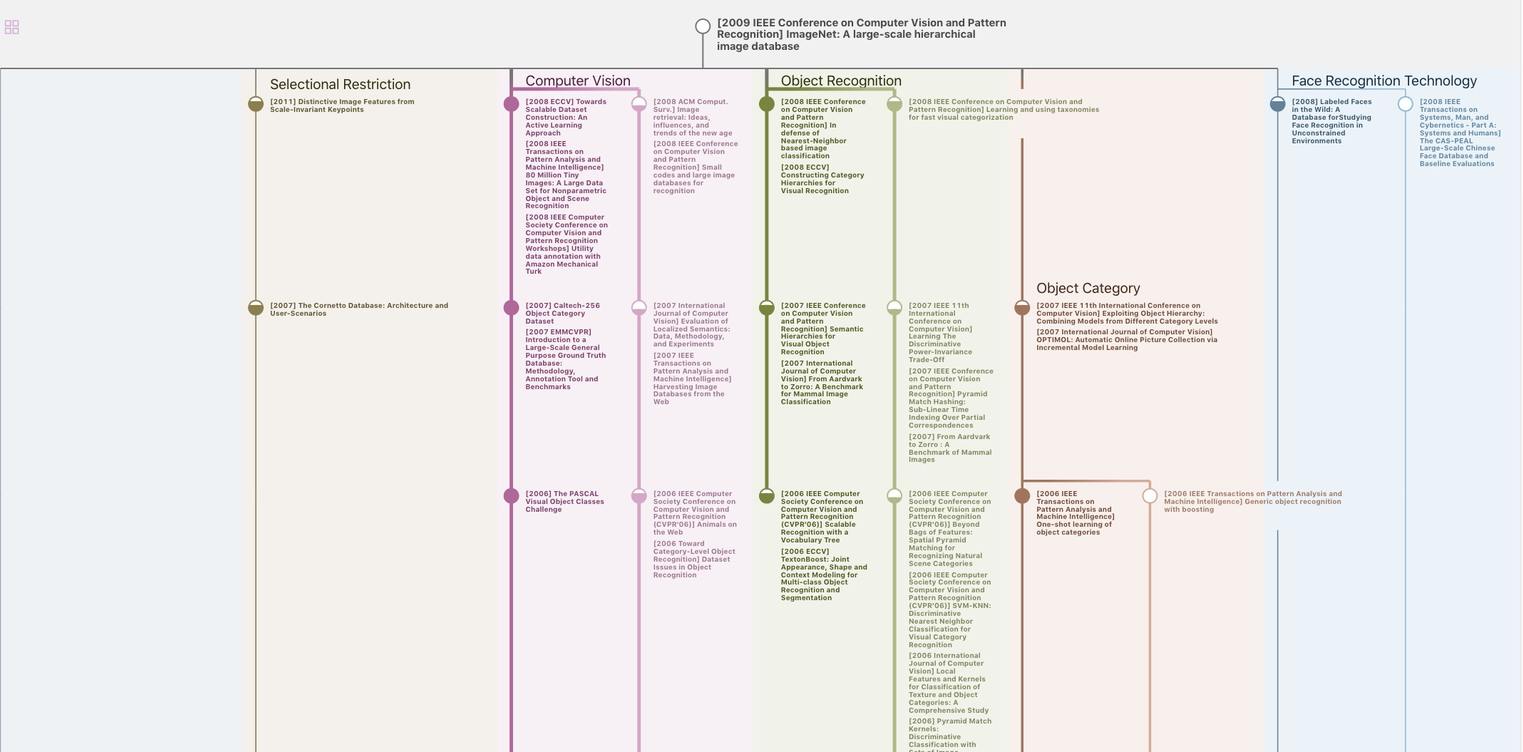
Generate MRT to find the research sequence of this paper
Chat Paper
Summary is being generated by the instructions you defined