Assessing the Modelling Approach and Datasets Required for Fault Detection in Photovoltaic Systems
2019 IEEE Industry Applications Society Annual Meeting(2019)
Key words
Fault detection,machine learning photovoltaics,random forest,weather data
AI Read Science
Must-Reading Tree
Example
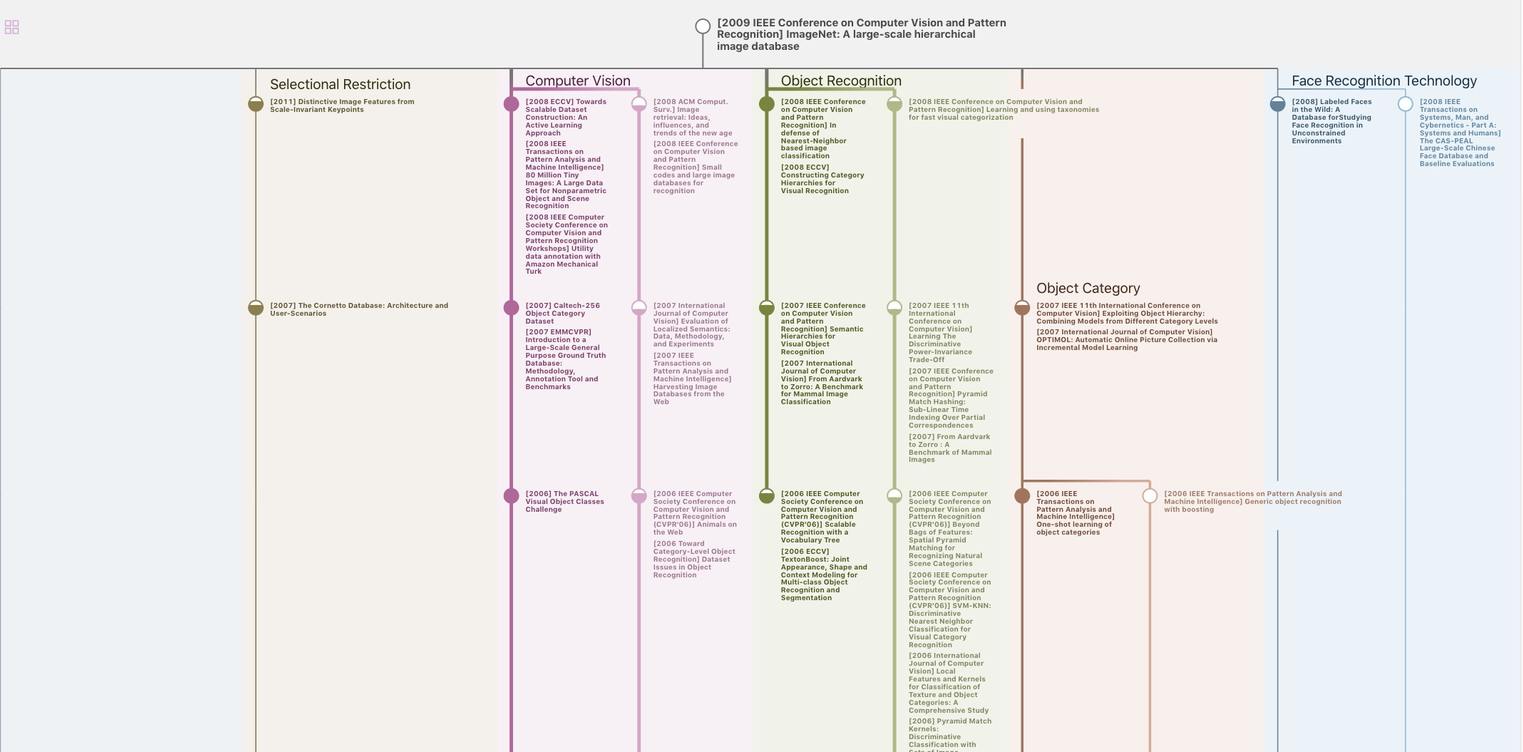
Generate MRT to find the research sequence of this paper
Chat Paper
Summary is being generated by the instructions you defined