Learning to Translate Between Real World and Simulated 3D Sensors While Transferring Task Models
2019 International Conference on 3D Vision (3DV)(2019)
摘要
Learning-based vision tasks are usually specialized on the sensor technology for which data has been labeled. The knowledge of a learned model is simply useless when it comes to data which differs from the data on which the model has been initially trained or if the model should be applied to a totally different imaging or sensor source. New labeled data has to be acquired on which a new model can be trained. Depending on the sensor, this can even get more complicated when the sensor data becomes more abstract and hard to be interpreted and labeled by humans. To enable reuse of models trained for a specific task across different sensors minimizes the data acquisition effort. Therefore, this work focuses on learning sensor models and translating between them, thus aiming for sensor interoperability. We show that even for the complex task of human pose estimation from 3D depth data recorded with different sensors, i.e. a simulated and a Kinect 2 TM depth sensor, human pose estimation can greatly improve by translating between sensor models without modifying the original task model. This process especially benefits sensors and applications for which labels and models are difficult if at all possible to retrieve from raw sensor data.
更多查看译文
关键词
Transfer Learning,Sensor Technologies,3D Sensing,Pose Recognition,Motion Capture,Domain Adaptation,Simulation,Character Animation
AI 理解论文
溯源树
样例
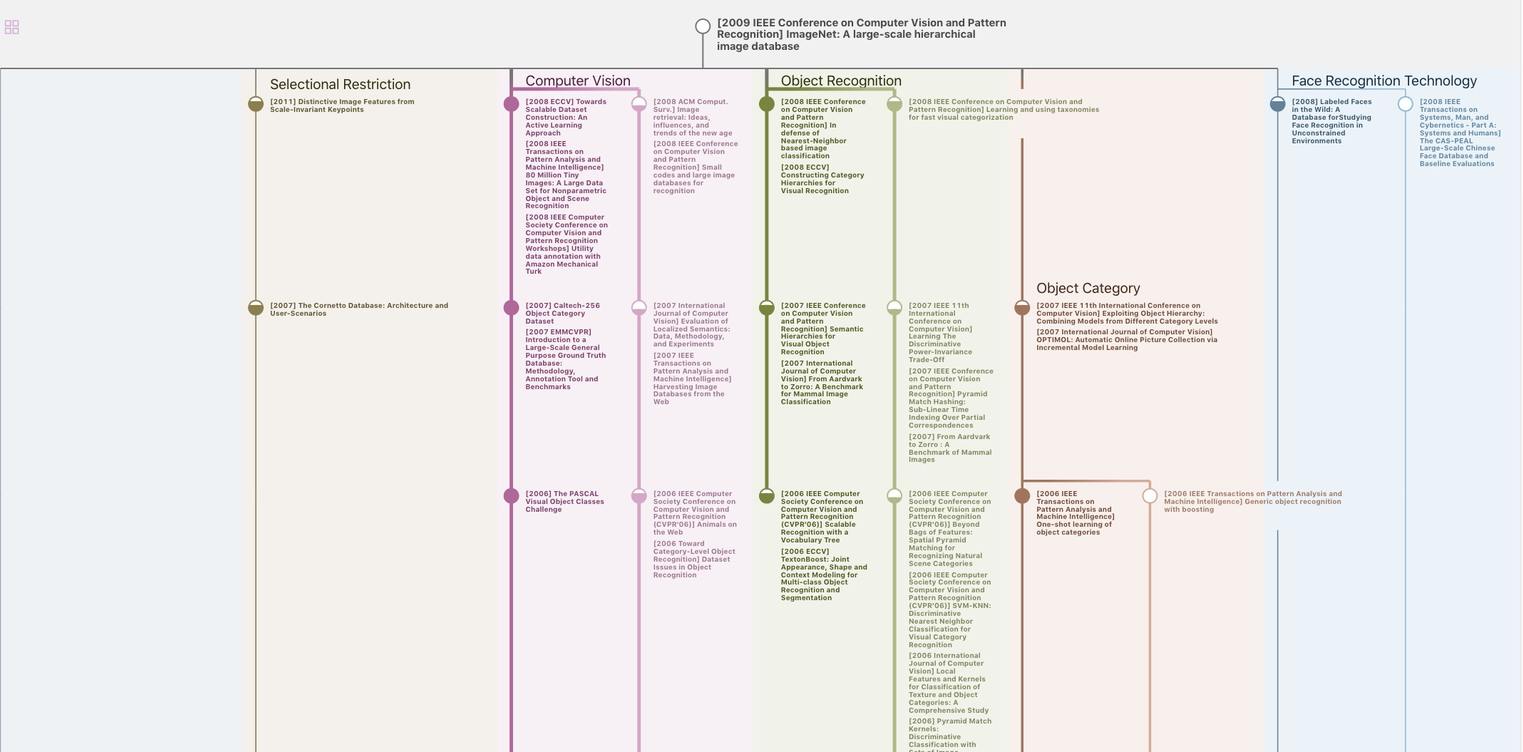
生成溯源树,研究论文发展脉络
Chat Paper
正在生成论文摘要