P2430Aortic shape synthesiser - understanding anatomical variations of the thoracic aorta
European Heart Journal(2019)
摘要
Abstract Introduction Population-based models of morphological variability are useful for automating medical image processing tasks, diagnosis, device and tool design, education and training as well as exploratory hypothesis formation in clinical research. We present a method to (a) generate such a model of the thoracic aorta, (b) explore the morphological characteristics of the underlying population in an intuitive visual and quantitative manner, and (c) correlate clinical parameters with morphological descriptors. With our approach one can synthesise new anatomically plausible aorta shapes, based on basic and intuitive parameter queries (e.g. shapes with a given stenosis degree and position). Methods We use a statistical shape model (SSM) as a compact mathematical representation of the geometric variability contained in a training population of 150 aortas of healthy, coarctation of the aorta and aortic valve disease patients. We describe each shape by centerlines of the aorta and supra aortic branches with inscribed radii at each centerline point. All shapes are registered with each other into a common shape space, where we compute a statistical mean as well as characteristic modes of variation. New shapes are synthesised by randomly sampling the shape modes and validated based on the underlying parameter distributions of the training data. This way we generate a representative database of anatomically plausible shapes. Querying the database with basic morphological parameters (e.g. aortic length, diameter, height-width ratio, stenosis size and position) returns a set of matching shapes based on the L1 norm on the given parameters. Results The first shape modes show that the greatest variance can be found in size, compactness, distance between supra-aortic branches and height-width ratio. In order to validate that the SSM represents the population well, 20 cases are left out when building it. It is then aligned to the unseen cases and the distance in coordinates and radii is evaluated. The matching error of only 1.54 mm in centerline coordinates and 0.16 mm in radii indicates that the SSM represents the population well. 1ehz748.07630 plausible shape instances are synthesised and queried with a set of 6 morphological parameters with a mean similarity score of 0.04. Application of the Kolmogorov-Smirnov statistics confirms that the morphological parameters of the synthesised and training shapes belong to the same distribution. Querying with a subset of parameters, e.g. a stenosis degree of 80 and stenosis position at the isthmus, allows to examine specific anatomical manifestations, see Fig. 1. Aortic shape synthesiser pipeline Conclusions The SSM methodology gives new insights into the correlation of morphological and clinical parameters within a given population. It allows to synthesise large numbers of representative new shapes, e.g. for data augmentation in artificial intelligence applications or large-scale functional analysis, e.g. via computational fluid dynamics simulation. Acknowledgement/Funding German Federal Ministry of Education and Research: “Medizintechnische Lösungen für die digitale Gesundheitsversorgung”
更多查看译文
AI 理解论文
溯源树
样例
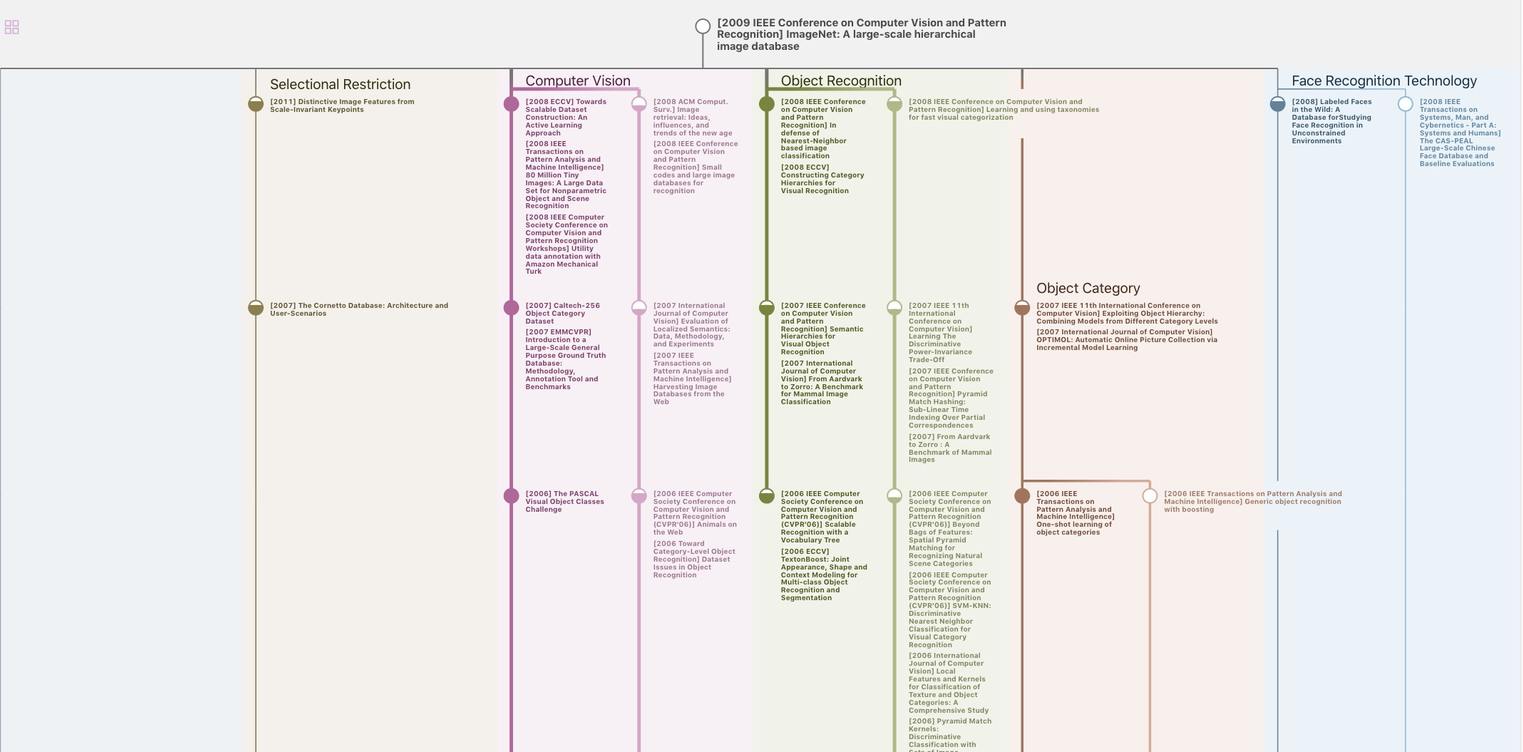
生成溯源树,研究论文发展脉络
Chat Paper
正在生成论文摘要