Compact Large-Aperture Fabry-Perot Interferometer Modules for Gas Spectroscopy at Mid-Ir
Proceedings of SPIE, the International Society for Optical Engineering/Proceedings of SPIE(2014)
关键词
Fabry-Perot Interferometer,gas,spectroscopy,infrared,correlation,tunable filter
AI 理解论文
溯源树
样例
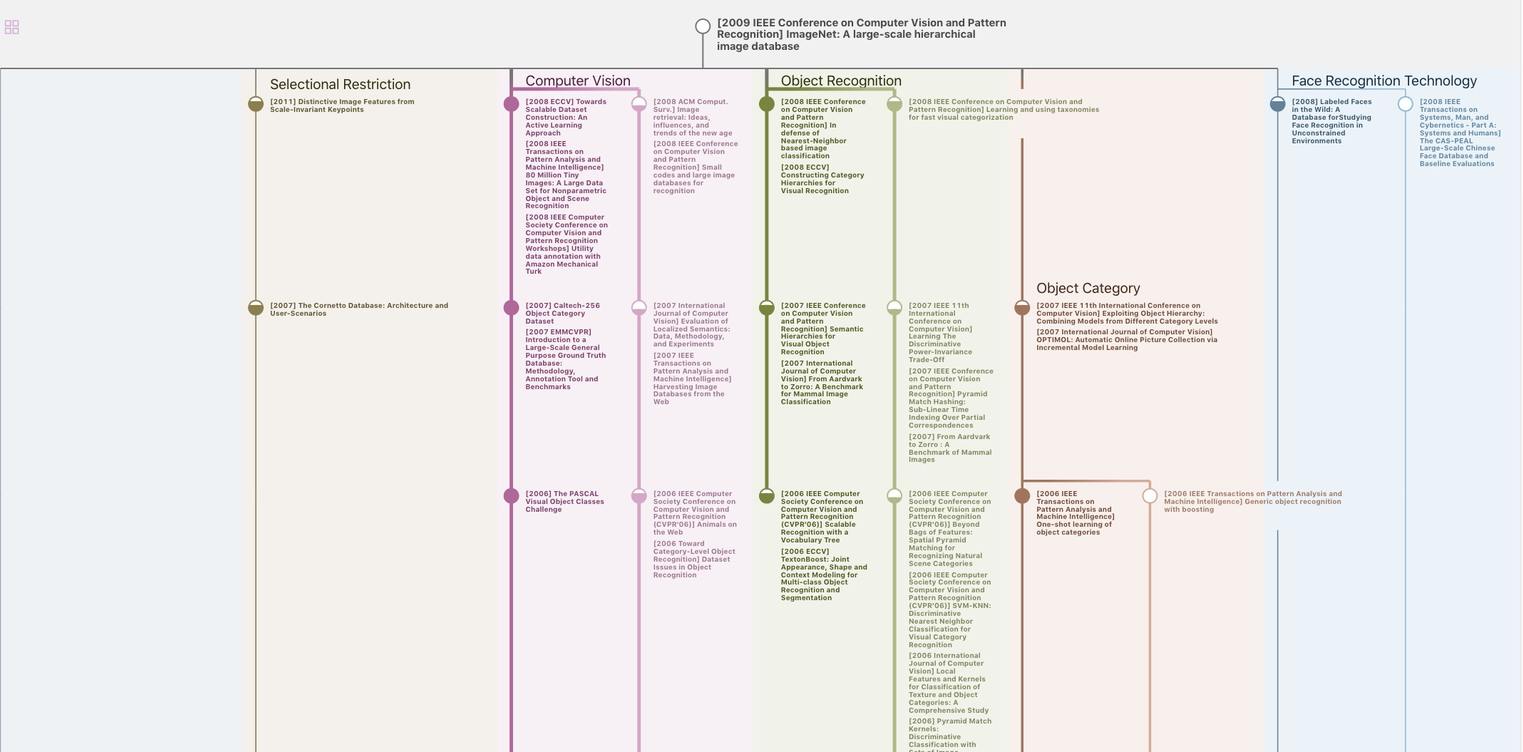
生成溯源树,研究论文发展脉络
Chat Paper
正在生成论文摘要