Progressive Unsupervised Person Re-Identification by Tracklet Association with Spatio-Temporal Regularization
IEEE transactions on multimedia(2021)
关键词
Cameras,Training,Feature extraction,Data models,Training data,Machine learning,UHDTV,Unsupervised person re-identification,Spatio-temporal regularization,Tracklet association
AI 理解论文
溯源树
样例
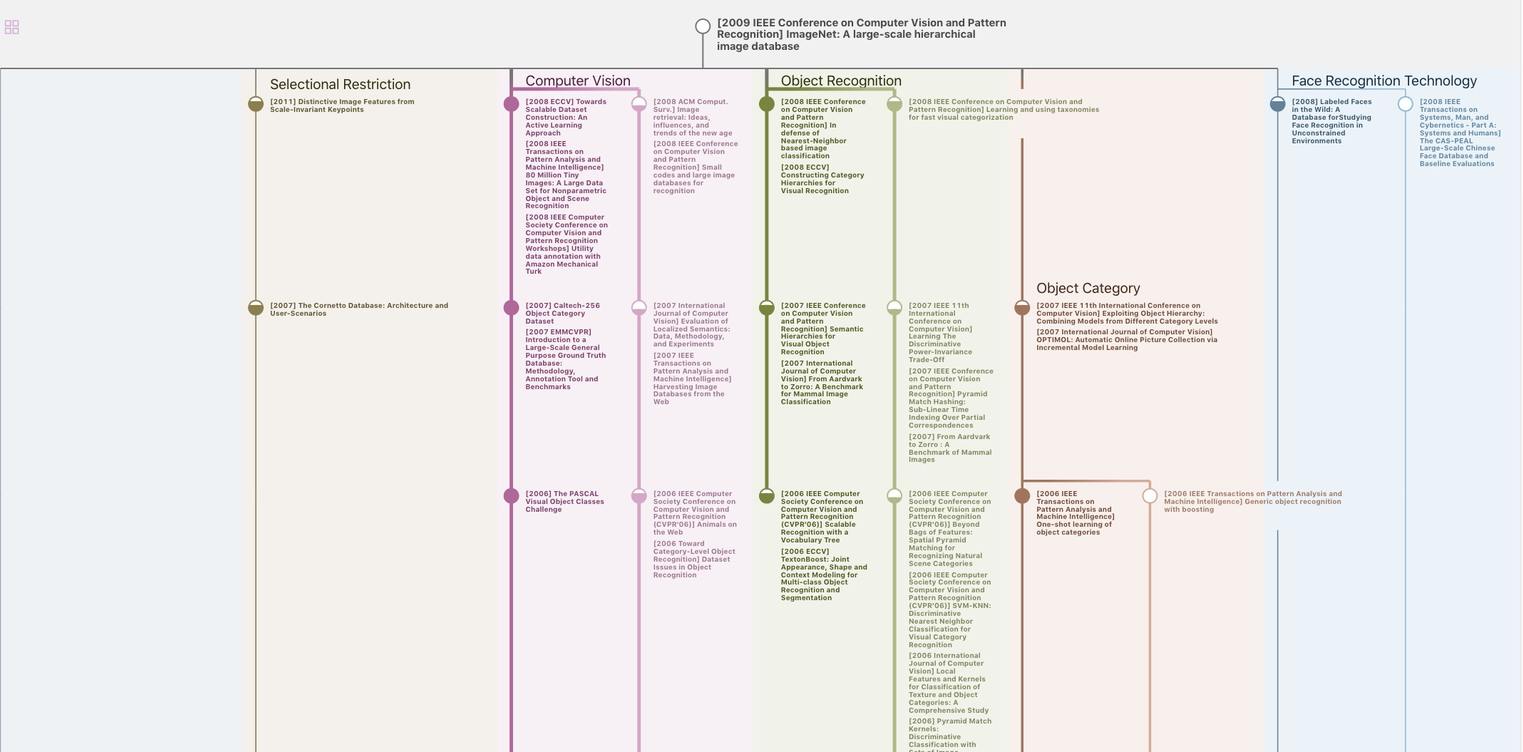
生成溯源树,研究论文发展脉络
Chat Paper
正在生成论文摘要