Exponential Ergodicity of the Bouncy Particle Sampler
The Annals of Statistics(2019)
关键词
Piecewise deterministic Markov process,Markov chain Monte Carlo,geometric ergodicity,change of variable,central limit theorem
AI 理解论文
溯源树
样例
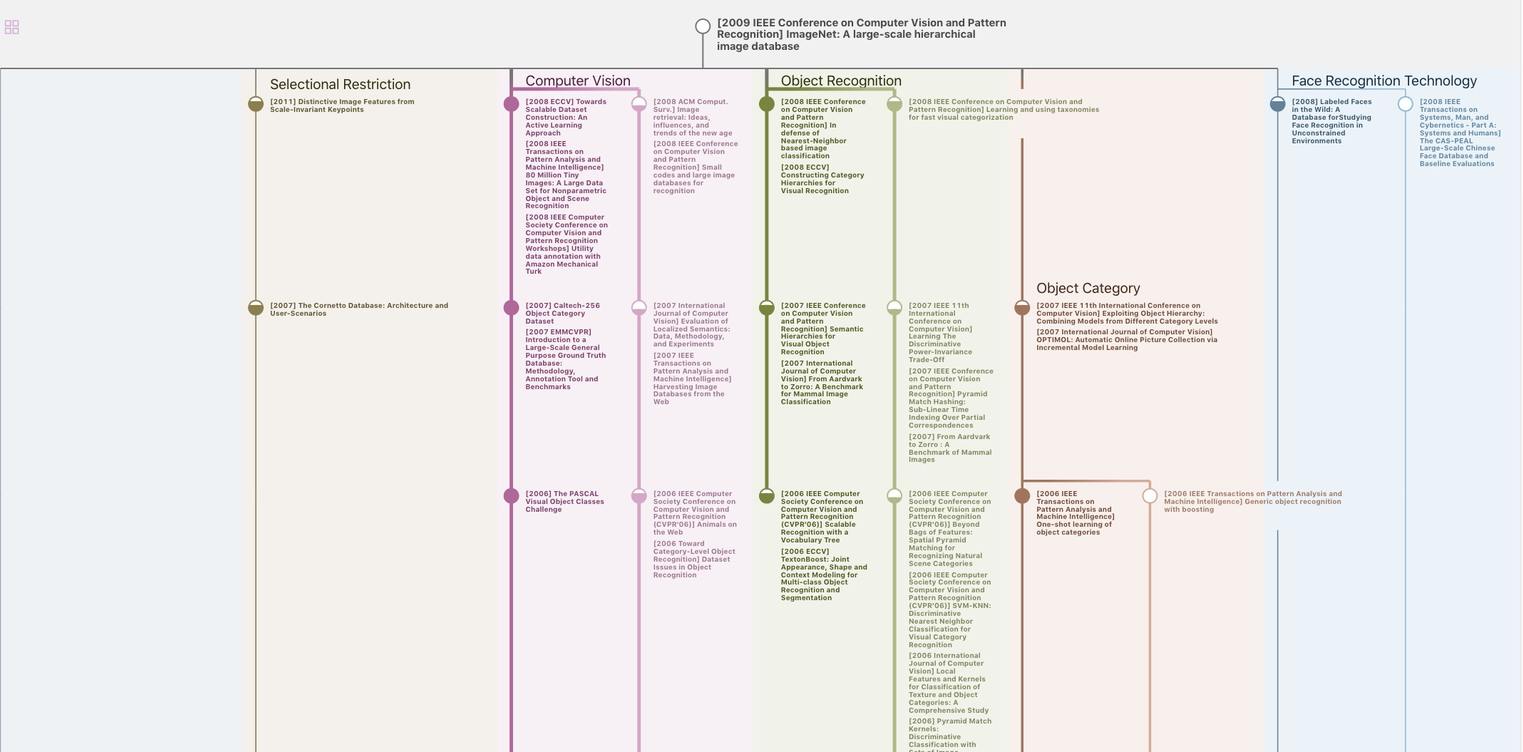
生成溯源树,研究论文发展脉络
Chat Paper
正在生成论文摘要