Detecting Suspects by Large-Scale Trajectory Patterns in the City
Mobile Information Systems(2019)
AI Read Science
Must-Reading Tree
Example
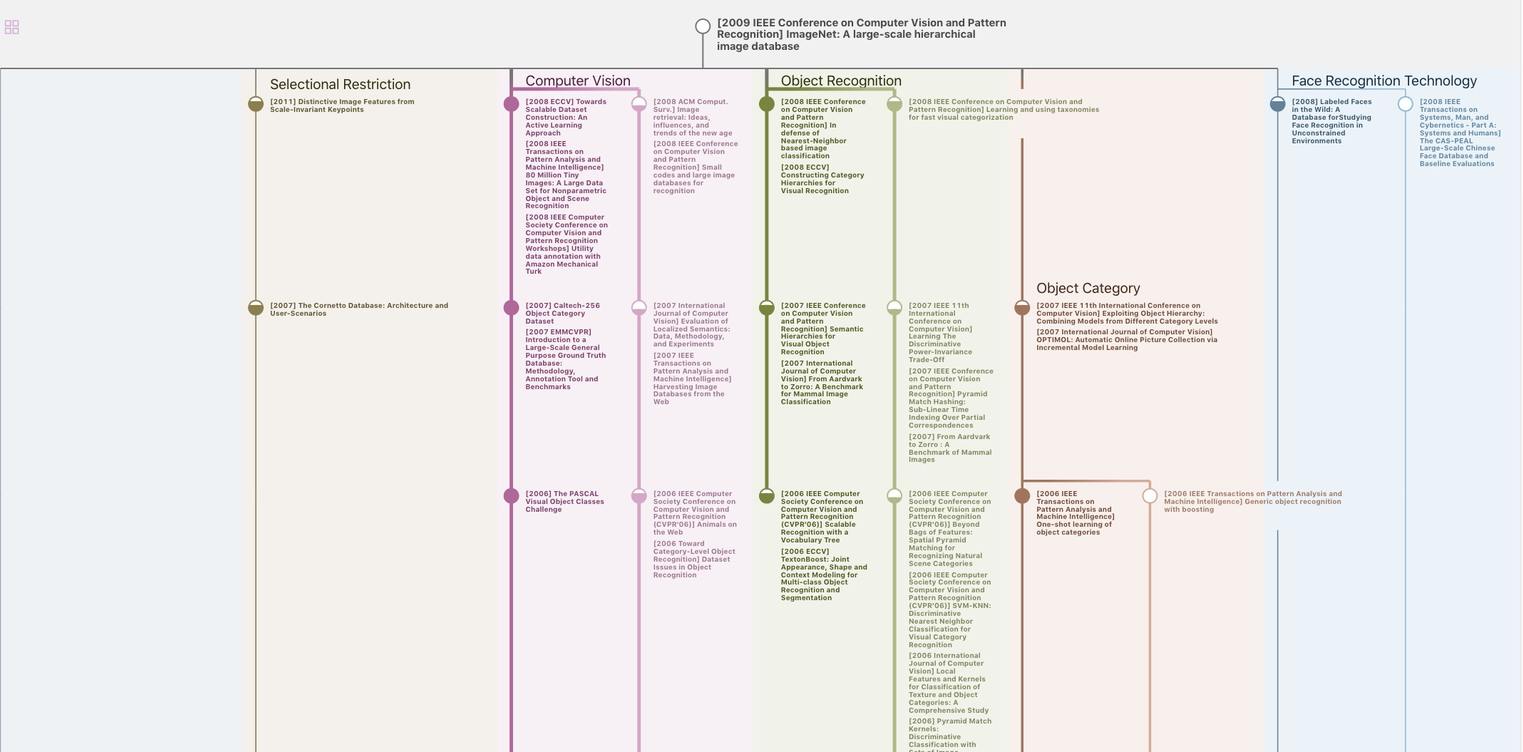
Generate MRT to find the research sequence of this paper
Chat Paper
Summary is being generated by the instructions you defined